el cat en
База данных: ELS EBSCO eBook
Page 3, Results: 38
Отмеченные записи: 0
21.
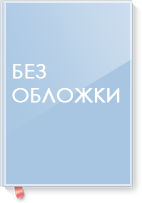
Подробнее
DDC 004.67/82
H 22
Handbook of research on cloud computing and big data applications in IoT / / B.B. Gupta and Dharma P. Agrawal, editors. - Hershey, PA : : Engineering Science Reference, , an imprint of IGI Global,, [2019]. - 1 online resource. - Includes bibliographical references. - URL: https://library.dvfu.ru/lib/document/SK_ELIB/33321ACE-D2FA-4917-8E33-BE52C3A179D4. - ISBN 9781522584087 (electronic bk.). - ISBN 1522584080 (electronic bk.)
Print version record.
Параллельные издания: Print version: : Handbook of research on cloud computing and big data applications in IoT. - Hershey, PA : Engineering Science Reference, , an imprint of IGI Global, [2019]. - ISBN 9781522584070
~РУБ DDC 004.67/82
Рубрики: Cloud computing.
Big data.
Internet of things.
Big data.
Cloud computing.
Internet of things.
COMPUTERS / Computer Literacy
COMPUTERS / Computer Science
COMPUTERS / Data Processing
COMPUTERS / Hardware / General
COMPUTERS / Information Technology
COMPUTERS / Machine Theory
COMPUTERS / Reference
Аннотация: "This book examines the latest research results on cloud computing and explores the broad applicability and scope of these trends on an international scale, venturing into the hot-button issue of IT services evolution and what we need to do to be prepared for future developments in cloud computing. It also explores big data applications in IoT"--
Доп.точки доступа:
Gupta, B. B., (1982-) \editor.\
H 22
Handbook of research on cloud computing and big data applications in IoT / / B.B. Gupta and Dharma P. Agrawal, editors. - Hershey, PA : : Engineering Science Reference, , an imprint of IGI Global,, [2019]. - 1 online resource. - Includes bibliographical references. - URL: https://library.dvfu.ru/lib/document/SK_ELIB/33321ACE-D2FA-4917-8E33-BE52C3A179D4. - ISBN 9781522584087 (electronic bk.). - ISBN 1522584080 (electronic bk.)
Print version record.
Параллельные издания: Print version: : Handbook of research on cloud computing and big data applications in IoT. - Hershey, PA : Engineering Science Reference, , an imprint of IGI Global, [2019]. - ISBN 9781522584070
Рубрики: Cloud computing.
Big data.
Internet of things.
Big data.
Cloud computing.
Internet of things.
COMPUTERS / Computer Literacy
COMPUTERS / Computer Science
COMPUTERS / Data Processing
COMPUTERS / Hardware / General
COMPUTERS / Information Technology
COMPUTERS / Machine Theory
COMPUTERS / Reference
Аннотация: "This book examines the latest research results on cloud computing and explores the broad applicability and scope of these trends on an international scale, venturing into the hot-button issue of IT services evolution and what we need to do to be prepared for future developments in cloud computing. It also explores big data applications in IoT"--
Доп.точки доступа:
Gupta, B. B., (1982-) \editor.\
22.
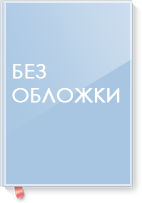
Подробнее
DDC 005.74
P 91
Prevos, Peter,.
Principles of strategic data science : : creating value from data, big and small / / Peter Prevos. - Birmingham, UK : : Packt Publishing Ltd.,, ©2019. - 1 online resource (vi, 85 pages). - URL: https://library.dvfu.ru/lib/document/SK_ELIB/FE754D04-C757-4754-8937-3B682727F7BE. - ISBN 9781838985509 (electronic book). - ISBN 1838985506 (electronic book)
Description based on online resource; title from digital title page (viewed on August 25, 2020).
Параллельные издания: Print version: : Prevos, Peter. Principles of Strategic Data Science : Creating Value from Data, Big and Small. - Birmingham : Packt Publishing, Limited, ©2019. - ISBN 9781838985295
Содержание:
Cover; FM; Table of Contents; Preface; Chapter 1: What is Data Science?; Introduction; Data-Driven Organization; The Data Revolution; The Elements of Data Science; Domain Knowledge; Mathematical Knowledge; Computer Science; The Unicorn Data Scientist?; The Purpose of Data Science; Chapter 2: Good Data Science; Introduction; A Data Science Trivium; Useful Data Science; Reality; Data; Information; Knowledge; The Feedback Loop; Sound Data Science; Validity; Reliability; Reproducibility; Governance; Aesthetic Data Science; Visualization; Reports; Best-Practice Data Science
Chapter 3: Strategic Data ScienceIntroduction; The Data Science Continuum; Collecting Data; Descriptive Statistics; Business Reporting; Diagnostics; Qualitative Data Science; Predicting the Future; Traditional Predictive Methods; Machine Learning; Prescribing Action; Toward a Data-Driven Organization; Chapter 4: The Data-Driven Organization; Introduction; People; The Data Science Team; Data Science Consumers; Data Science Culture; Systems; Process; Define; Prepare; Understand; Communicate; The Limitations of Data Science; The Limits of Computation; Ethical Data Science; Chapter 5: References; Index.
~РУБ DDC 005.74
Рубрики: Databases.
Data mining.
Big data.
Electronic data processing.
Big data.
Data mining.
Databases.
Electronic data processing.
Аннотация: Principles of Strategic Data Science describes a framework that creates value from data to help organizations meet their objectives. With this book, you'll bridge the gap between mathematics and computer science and also gain insight into the workings of the entire data science pipeline.
P 91
Prevos, Peter,.
Principles of strategic data science : : creating value from data, big and small / / Peter Prevos. - Birmingham, UK : : Packt Publishing Ltd.,, ©2019. - 1 online resource (vi, 85 pages). - URL: https://library.dvfu.ru/lib/document/SK_ELIB/FE754D04-C757-4754-8937-3B682727F7BE. - ISBN 9781838985509 (electronic book). - ISBN 1838985506 (electronic book)
Description based on online resource; title from digital title page (viewed on August 25, 2020).
Параллельные издания: Print version: : Prevos, Peter. Principles of Strategic Data Science : Creating Value from Data, Big and Small. - Birmingham : Packt Publishing, Limited, ©2019. - ISBN 9781838985295
Содержание:
Cover; FM; Table of Contents; Preface; Chapter 1: What is Data Science?; Introduction; Data-Driven Organization; The Data Revolution; The Elements of Data Science; Domain Knowledge; Mathematical Knowledge; Computer Science; The Unicorn Data Scientist?; The Purpose of Data Science; Chapter 2: Good Data Science; Introduction; A Data Science Trivium; Useful Data Science; Reality; Data; Information; Knowledge; The Feedback Loop; Sound Data Science; Validity; Reliability; Reproducibility; Governance; Aesthetic Data Science; Visualization; Reports; Best-Practice Data Science
Chapter 3: Strategic Data ScienceIntroduction; The Data Science Continuum; Collecting Data; Descriptive Statistics; Business Reporting; Diagnostics; Qualitative Data Science; Predicting the Future; Traditional Predictive Methods; Machine Learning; Prescribing Action; Toward a Data-Driven Organization; Chapter 4: The Data-Driven Organization; Introduction; People; The Data Science Team; Data Science Consumers; Data Science Culture; Systems; Process; Define; Prepare; Understand; Communicate; The Limitations of Data Science; The Limits of Computation; Ethical Data Science; Chapter 5: References; Index.
Рубрики: Databases.
Data mining.
Big data.
Electronic data processing.
Big data.
Data mining.
Databases.
Electronic data processing.
Аннотация: Principles of Strategic Data Science describes a framework that creates value from data to help organizations meet their objectives. With this book, you'll bridge the gap between mathematics and computer science and also gain insight into the workings of the entire data science pipeline.
23.
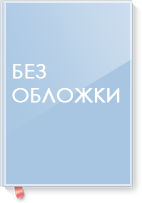
Подробнее
DDC 006.3/12
E 50
Emerging perspectives in big data warehousing / / David Taniar and Wenny Rahayu [editors]. - 4018/978-1-5225-5516-2. - Hershey, PA : : IGI Global,, [2019]. - 1 online resource (xviii, 348 pages) ( час. мин.), 4018/978-1-5225-5516-2. - Includes bibliographical references and index. - URL: https://library.dvfu.ru/lib/document/SK_ELIB/2F057049-6875-4EB8-9D1B-FFADAC1B66E4. - ISBN 152255517X. - ISBN 9781522555179 (electronic book)
Description based on online resource; title from digital title page (viewed on June 27, 2019).
Параллельные издания: Print version: :
Содержание:
Chapter 1. A two-tiered segmentation approach for transaction data warehousing -- Chapter 2. Real-time big data warehousing -- Chapter 3. Deductive data warehouses: analyzing data warehouses with datalog (by example) -- Chapter 4. A trajectory ontology design pattern for semantic trajectory data warehouses: behavior analysis and animal tracking case studies -- Chapter 5. Personalized spatio-temporal OLAP queries suggestion based on user behavior and a new similarity measure -- Chapter 6. Building OLAP cubes from columnar NoSQL data warehouses -- Chapter 7. Commercial and open source business intelligence platforms for big data warehousing -- Chapter 8. Index structures for data warehousing and big data analytics -- Chapter 9. Multidimensional analysis of big data -- Chapter 10. Development of ETL processes using the domain-specific modeling approach -- Chapter 11. Introduction of item constraints to discover characteristic sequential patterns.
~РУБ DDC 006.3/12
Рубрики: Data warehousing.
Data mining.
Big data.
COMPUTERS / General
Аннотация: "This book disseminates the latest research findings in the areas of data management and analyzation. It also focuses on the integration between the fields of data warehousing and data mining"--
Доп.точки доступа:
Taniar, David, \editor.\
Rahayu, Johanna Wenny, \editor.\
IGI Global,
E 50
Emerging perspectives in big data warehousing / / David Taniar and Wenny Rahayu [editors]. - 4018/978-1-5225-5516-2. - Hershey, PA : : IGI Global,, [2019]. - 1 online resource (xviii, 348 pages) ( час. мин.), 4018/978-1-5225-5516-2. - Includes bibliographical references and index. - URL: https://library.dvfu.ru/lib/document/SK_ELIB/2F057049-6875-4EB8-9D1B-FFADAC1B66E4. - ISBN 152255517X. - ISBN 9781522555179 (electronic book)
Description based on online resource; title from digital title page (viewed on June 27, 2019).
Параллельные издания: Print version: :
Содержание:
Chapter 1. A two-tiered segmentation approach for transaction data warehousing -- Chapter 2. Real-time big data warehousing -- Chapter 3. Deductive data warehouses: analyzing data warehouses with datalog (by example) -- Chapter 4. A trajectory ontology design pattern for semantic trajectory data warehouses: behavior analysis and animal tracking case studies -- Chapter 5. Personalized spatio-temporal OLAP queries suggestion based on user behavior and a new similarity measure -- Chapter 6. Building OLAP cubes from columnar NoSQL data warehouses -- Chapter 7. Commercial and open source business intelligence platforms for big data warehousing -- Chapter 8. Index structures for data warehousing and big data analytics -- Chapter 9. Multidimensional analysis of big data -- Chapter 10. Development of ETL processes using the domain-specific modeling approach -- Chapter 11. Introduction of item constraints to discover characteristic sequential patterns.
Рубрики: Data warehousing.
Data mining.
Big data.
COMPUTERS / General
Аннотация: "This book disseminates the latest research findings in the areas of data management and analyzation. It also focuses on the integration between the fields of data warehousing and data mining"--
Доп.точки доступа:
Taniar, David, \editor.\
Rahayu, Johanna Wenny, \editor.\
IGI Global,
24.
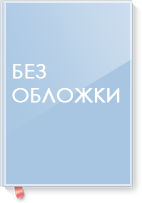
Подробнее
DDC 519.5
H 22
Hand, (David J.), (1950-).
Dark data : : why what you don't know matters / / David J. Hand. - Princeton : : Princeton University Press,, [2020]. - 1 online resource. - Includes bibliographical references and index. - URL: https://library.dvfu.ru/lib/document/SK_ELIB/CB030912-F809-4C70-92A8-3E5AADF22754. - ISBN 0691198853. - ISBN 9780691198859 (electronic bk.)
Description based on print version record and CIP data provided by publisher; resource not viewed.
Параллельные издания: Print version: : Hand, D. J. (David J.), 1950- Dark data. - Princeton : Princeton University Press, [2020]. - ISBN 9780691182377
Содержание:
Cover; Contents; Preface; Part 1: Dark Data: Their Origins and Consequences; Chapter 1: Dark Data: What We Don't See Shapes Our World; The Ghost of Data; So You Think You Have All the Data?; Nothing Happened, So We Ignored It; The Power of Dark Data; All around Us; Chapter 2: Discovering Dark Data: What We Collect and What We Don't; Dark Data on All Sides; Data Exhaust, Selection, and Self-Selection; From the Few to the Many; Experimental Data; Beware Human Frailties; Chapter 3: Definitions and Dark Data: What Do You Want to Know?; Different Definitions and Measuring the Wrong Thing
You Can't Measure EverythingScreening; Selection on the Basis of Past Performance; Chapter 4: Unintentional Dark Data: Saying One Thing, Doing Another; The Big Picture; Summarizing; Human Error; Instrument Limitations; Linking Data Sets; Chapter 5: Strategic Dark Data: Gaming, Feedback, and Information Asymmetry; Gaming; Feedback; Information Asymmetry; Adverse Selection and Algorithms; Chapter 6: Intentional Dark Data: Fraud and Deception; Fraud; Identity Theft and Internet Fraud; Personal Financial Fraud; Financial Market Fraud and Insider Trading; Insurance Fraud; And More
Chapter 7: Science and Dark Data: The Nature of DiscoveryThe Nature of Science; If Only I'd Known That; Tripping over Dark Data; Dark Data and the Big Picture; Hiding the Facts; Retraction; Provenance and Trustworthiness: Who Told You That?; Part II: Illuminating and Using Dark Data; Chapter 8: Dealing with Dark Data: Shining a Light; Hope!; Linking Observed and Missing Data; Identifying the Missing Data Mechanism; Working with the Data We Have; Going Beyond the Data: What If You Die First?; Going Beyond the Data: Imputation; Iteration; Wrong Number!
Chapter 9: Benefiting from Dark Data: Reframing the QuestionHiding Data; Hiding Data from Ourselves: Randomized Controlled Trials; What Might Have Been; Replicated Data; Imaginary Data: The Bayesian Prior; Privacy and Confidentiality Preservation; Collecting Data in the Dark; Chapter 10: Classifying Dark Data: A Route through the Maze; A Taxonomy of Dark Data; Illumination; Notes; Index
~РУБ DDC 519.5
Рубрики: Missing observations (Statistics)
Big data.
Big data.
Missing observations (Statistics)
COMPUTERS / Database Management / Data Mining
Аннотация: "Data describe and represent the world. However, no matter how big they may be, data sets don't - indeed cannot - capture everything. Data are measurements - and, as such, they represent only what has been measured. They don't necessarily capture all the information that is relevant to the questions we may want to ask. If we do not take into account what may be missing/unknown in the data we have, we may find ourselves unwittingly asking questions that our data cannot actually address, come to mistaken conclusions, and make disastrous decisions. In this book, David Hand looks at the ubiquitous phenomenon of "missing data." He calls this "dark data" (making a comparison to "dark matter" - i.e., matter in the universe that we know is there, but which is invisible to direct measurement). He reveals how we can detect when data is missing, the types of settings in which missing data are likely to be found, and what to do about it. It can arise for many reasons, which themselves may not be obvious - for example, asymmetric information in wars; time delays in financial trading; dropouts in clinical trials; deliberate selection to enhance apparent performance in hospitals, policing, and schools; etc. What becomes clear is that measuring and collecting more and more data (big data) will not necessarily lead us to better understanding or to better decisions. We need to be vigilant to what is missing or unknown in our data, so that we can try to control for it. How do we do that? We can be alert to the causes of dark data, design better data-collection strategies that sidestep some of these causes - and, we can ask better questions of our data, which will lead us to deeper insights and better decisions"--
H 22
Hand, (David J.), (1950-).
Dark data : : why what you don't know matters / / David J. Hand. - Princeton : : Princeton University Press,, [2020]. - 1 online resource. - Includes bibliographical references and index. - URL: https://library.dvfu.ru/lib/document/SK_ELIB/CB030912-F809-4C70-92A8-3E5AADF22754. - ISBN 0691198853. - ISBN 9780691198859 (electronic bk.)
Description based on print version record and CIP data provided by publisher; resource not viewed.
Параллельные издания: Print version: : Hand, D. J. (David J.), 1950- Dark data. - Princeton : Princeton University Press, [2020]. - ISBN 9780691182377
Содержание:
Cover; Contents; Preface; Part 1: Dark Data: Their Origins and Consequences; Chapter 1: Dark Data: What We Don't See Shapes Our World; The Ghost of Data; So You Think You Have All the Data?; Nothing Happened, So We Ignored It; The Power of Dark Data; All around Us; Chapter 2: Discovering Dark Data: What We Collect and What We Don't; Dark Data on All Sides; Data Exhaust, Selection, and Self-Selection; From the Few to the Many; Experimental Data; Beware Human Frailties; Chapter 3: Definitions and Dark Data: What Do You Want to Know?; Different Definitions and Measuring the Wrong Thing
You Can't Measure EverythingScreening; Selection on the Basis of Past Performance; Chapter 4: Unintentional Dark Data: Saying One Thing, Doing Another; The Big Picture; Summarizing; Human Error; Instrument Limitations; Linking Data Sets; Chapter 5: Strategic Dark Data: Gaming, Feedback, and Information Asymmetry; Gaming; Feedback; Information Asymmetry; Adverse Selection and Algorithms; Chapter 6: Intentional Dark Data: Fraud and Deception; Fraud; Identity Theft and Internet Fraud; Personal Financial Fraud; Financial Market Fraud and Insider Trading; Insurance Fraud; And More
Chapter 7: Science and Dark Data: The Nature of DiscoveryThe Nature of Science; If Only I'd Known That; Tripping over Dark Data; Dark Data and the Big Picture; Hiding the Facts; Retraction; Provenance and Trustworthiness: Who Told You That?; Part II: Illuminating and Using Dark Data; Chapter 8: Dealing with Dark Data: Shining a Light; Hope!; Linking Observed and Missing Data; Identifying the Missing Data Mechanism; Working with the Data We Have; Going Beyond the Data: What If You Die First?; Going Beyond the Data: Imputation; Iteration; Wrong Number!
Chapter 9: Benefiting from Dark Data: Reframing the QuestionHiding Data; Hiding Data from Ourselves: Randomized Controlled Trials; What Might Have Been; Replicated Data; Imaginary Data: The Bayesian Prior; Privacy and Confidentiality Preservation; Collecting Data in the Dark; Chapter 10: Classifying Dark Data: A Route through the Maze; A Taxonomy of Dark Data; Illumination; Notes; Index
Рубрики: Missing observations (Statistics)
Big data.
Big data.
Missing observations (Statistics)
COMPUTERS / Database Management / Data Mining
Аннотация: "Data describe and represent the world. However, no matter how big they may be, data sets don't - indeed cannot - capture everything. Data are measurements - and, as such, they represent only what has been measured. They don't necessarily capture all the information that is relevant to the questions we may want to ask. If we do not take into account what may be missing/unknown in the data we have, we may find ourselves unwittingly asking questions that our data cannot actually address, come to mistaken conclusions, and make disastrous decisions. In this book, David Hand looks at the ubiquitous phenomenon of "missing data." He calls this "dark data" (making a comparison to "dark matter" - i.e., matter in the universe that we know is there, but which is invisible to direct measurement). He reveals how we can detect when data is missing, the types of settings in which missing data are likely to be found, and what to do about it. It can arise for many reasons, which themselves may not be obvious - for example, asymmetric information in wars; time delays in financial trading; dropouts in clinical trials; deliberate selection to enhance apparent performance in hospitals, policing, and schools; etc. What becomes clear is that measuring and collecting more and more data (big data) will not necessarily lead us to better understanding or to better decisions. We need to be vigilant to what is missing or unknown in our data, so that we can try to control for it. How do we do that? We can be alert to the causes of dark data, design better data-collection strategies that sidestep some of these causes - and, we can ask better questions of our data, which will lead us to deeper insights and better decisions"--
25.
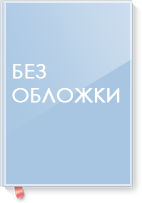
Подробнее
DDC 005.7
B 57
Big data analytics for sustainable computing / / [edited by] Anandakumar Haldorai, Arulmurugan Ramu. - 4018/978-1-5225-9750-6. - Hershey, Pennsylvania : : IGI Global,, [2020]. - 1 online resource. ( час. мин.), 4018/978-1-5225-9750-6. - (Advances in Data Mining and Database Management (ADMDM) Book Series). - Includes bibliographical references and index. - URL: https://library.dvfu.ru/lib/document/SK_ELIB/3D8B2ECA-E6CC-4BC1-BA05-C785DB65E243. - ISBN 9781522597520 (electronic book). - ISBN 1522597522 (electronic book)
Description based on online resource; title from digital title page (viewed on December 02, 2019).
Параллельные издания: Print version: :
Содержание:
Chapter 1. Understanding big data -- Chapter 2. A detailed study on classification algorithms in big data -- Chapter 3. Big data and analytics -- Chapter 4. Decoding big data analytics for emerging business through data-intensive applications and business intelligence: a review on analytics applications and theoretical aspects -- Chapter 5. Feature selection algorithm using relative odds for data mining classification -- Chapter 6. Social network analysis -- Chapter 7. Role of machine intelligence and big data in remote sensing -- Chapter 8. Provisioning system for application virtualization environments -- Chapter 9. Big data-based spectrum sensing for cognitive radio networks using artificial intelligence -- Chapter 10. Big data analytics in the healthcare industry: an analysis of healthcare applications in machine learning with big data analytics -- Chapter 11. Big data analytics and visualization for food health status determination using bigmart data -- Chapter 12. "Saksham model" performance improvisation using Node capability evaluation in apache hadoop.
~РУБ DDC 005.7
Рубрики: Big data.
Big data.
Аннотация: Big data consists of data sets that are too large and complex for traditional data processing and data management applications. Therefore, to obtain the valuable information within the data, one must use a variety of innovative analytical methods, such as web analytics, machine learning, and network analytics. As the study of big data becomes more popular, there is an urgent demand for studies on high-level computational intelligence and computing services for analyzing this significant area of information science. Big Data Analytics for Sustainable Computing is a collection of innovative rese.
Доп.точки доступа:
Haldorai, Anandakumar, (1983-) \editor.\
Ramu, Arulmurugan, (1985-) \editor.\
IGI Global,
B 57
Big data analytics for sustainable computing / / [edited by] Anandakumar Haldorai, Arulmurugan Ramu. - 4018/978-1-5225-9750-6. - Hershey, Pennsylvania : : IGI Global,, [2020]. - 1 online resource. ( час. мин.), 4018/978-1-5225-9750-6. - (Advances in Data Mining and Database Management (ADMDM) Book Series). - Includes bibliographical references and index. - URL: https://library.dvfu.ru/lib/document/SK_ELIB/3D8B2ECA-E6CC-4BC1-BA05-C785DB65E243. - ISBN 9781522597520 (electronic book). - ISBN 1522597522 (electronic book)
Description based on online resource; title from digital title page (viewed on December 02, 2019).
Параллельные издания: Print version: :
Содержание:
Chapter 1. Understanding big data -- Chapter 2. A detailed study on classification algorithms in big data -- Chapter 3. Big data and analytics -- Chapter 4. Decoding big data analytics for emerging business through data-intensive applications and business intelligence: a review on analytics applications and theoretical aspects -- Chapter 5. Feature selection algorithm using relative odds for data mining classification -- Chapter 6. Social network analysis -- Chapter 7. Role of machine intelligence and big data in remote sensing -- Chapter 8. Provisioning system for application virtualization environments -- Chapter 9. Big data-based spectrum sensing for cognitive radio networks using artificial intelligence -- Chapter 10. Big data analytics in the healthcare industry: an analysis of healthcare applications in machine learning with big data analytics -- Chapter 11. Big data analytics and visualization for food health status determination using bigmart data -- Chapter 12. "Saksham model" performance improvisation using Node capability evaluation in apache hadoop.
Рубрики: Big data.
Big data.
Аннотация: Big data consists of data sets that are too large and complex for traditional data processing and data management applications. Therefore, to obtain the valuable information within the data, one must use a variety of innovative analytical methods, such as web analytics, machine learning, and network analytics. As the study of big data becomes more popular, there is an urgent demand for studies on high-level computational intelligence and computing services for analyzing this significant area of information science. Big Data Analytics for Sustainable Computing is a collection of innovative rese.
Доп.точки доступа:
Haldorai, Anandakumar, (1983-) \editor.\
Ramu, Arulmurugan, (1985-) \editor.\
IGI Global,
26.
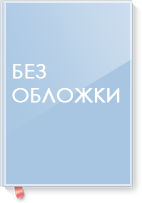
Подробнее
DDC 006.3/12
D 30
Deep learning : : concepts, methodologies, tools, and applications / / Information Resources Management Association. - Hershey, PA : : Engineering Science Reference, an imprint of IGI Global,, [2020]. - 1 online resource. - Includes bibliographical references and index. - URL: https://library.dvfu.ru/lib/document/SK_ELIB/B006D724-0D0B-4DBC-9027-6261FB49F604. - ISBN 1799804151 (electronic book). - ISBN 9781799804161 (electronic book). - ISBN 179980416X (electronic book). - ISBN 9781799804154 (electronic bk.)
Description based on online resource; title from digital title page (viewed on November 26, 2019).
Параллельные издания: Print version: : Deep learning (Engineering Science Reference (Publisher)) Deep learning. - Hershey : Engineering Science Reference, 2019. - ISBN 9781799804147
~РУБ DDC 006.3/12
Рубрики: Data mining.
Machine learning--Industrial applications.
Big data.
Invisible Web.
Big data.
Data mining.
Invisible Web.
Machine learning--Industrial applications.
Аннотация: "This book is a vital reference source that trends in data analytics and potential technologies that will facilitate insight in various domains of science, industry, business, and consumer applications. It also explores the latest concepts, algorithms, and techniques of deep learning and data mining and analysis"--
Доп.точки доступа:
Information Resources Management Association,
D 30
Deep learning : : concepts, methodologies, tools, and applications / / Information Resources Management Association. - Hershey, PA : : Engineering Science Reference, an imprint of IGI Global,, [2020]. - 1 online resource. - Includes bibliographical references and index. - URL: https://library.dvfu.ru/lib/document/SK_ELIB/B006D724-0D0B-4DBC-9027-6261FB49F604. - ISBN 1799804151 (electronic book). - ISBN 9781799804161 (electronic book). - ISBN 179980416X (electronic book). - ISBN 9781799804154 (electronic bk.)
Description based on online resource; title from digital title page (viewed on November 26, 2019).
Параллельные издания: Print version: : Deep learning (Engineering Science Reference (Publisher)) Deep learning. - Hershey : Engineering Science Reference, 2019. - ISBN 9781799804147
Рубрики: Data mining.
Machine learning--Industrial applications.
Big data.
Invisible Web.
Big data.
Data mining.
Invisible Web.
Machine learning--Industrial applications.
Аннотация: "This book is a vital reference source that trends in data analytics and potential technologies that will facilitate insight in various domains of science, industry, business, and consumer applications. It also explores the latest concepts, algorithms, and techniques of deep learning and data mining and analysis"--
Доп.точки доступа:
Information Resources Management Association,
27.
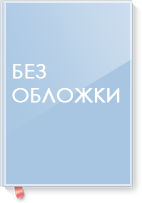
Подробнее
DDC 005.7
D 30
Deep learning techniques and optimization strategies in big data analytics / / [edited by] J. Joshua Thomas, Pinar Karagoz, B. Bazeer Ahamed, Pandian Vasant. - Hershey, PA : : Engineering Science Reference, IGI Global,, [2020]. - 1 online resource (xxv, 355 pages). : il. - (Advances in Systems Analysis, Software Engineering, and High Performance Computing (ASASEHPC) Book Series). - Includes bibliographical references and index. - URL: https://library.dvfu.ru/lib/document/SK_ELIB/DACCE85B-FCAD-4E91-B9ED-BAE1B2C07407. - ISBN 1799811948 (electronic book). - ISBN 9781799811947 (electronic bk.)
Description based on online resource; title from digital title page (viewed on December 02, 2019).
Параллельные издания: Print version: : Deep learning techniques and optimization strategies in big data analytics. - Hershey, PA : Engineering Science Reference, [2020]. - ISBN 9781799811923
~РУБ DDC 005.7
Рубрики: Big data.
Quantitative research.
Big data.
Quantitative research.
Аннотация: "This book examines the application of artificial intelligence in machine learning, data mining in unstructured data sets or databases, web mining, and information retrieval"--
Доп.точки доступа:
Thomas, J. Joshua, (1973-) \editor.\
D 30
Deep learning techniques and optimization strategies in big data analytics / / [edited by] J. Joshua Thomas, Pinar Karagoz, B. Bazeer Ahamed, Pandian Vasant. - Hershey, PA : : Engineering Science Reference, IGI Global,, [2020]. - 1 online resource (xxv, 355 pages). : il. - (Advances in Systems Analysis, Software Engineering, and High Performance Computing (ASASEHPC) Book Series). - Includes bibliographical references and index. - URL: https://library.dvfu.ru/lib/document/SK_ELIB/DACCE85B-FCAD-4E91-B9ED-BAE1B2C07407. - ISBN 1799811948 (electronic book). - ISBN 9781799811947 (electronic bk.)
Description based on online resource; title from digital title page (viewed on December 02, 2019).
Параллельные издания: Print version: : Deep learning techniques and optimization strategies in big data analytics. - Hershey, PA : Engineering Science Reference, [2020]. - ISBN 9781799811923
Рубрики: Big data.
Quantitative research.
Big data.
Quantitative research.
Аннотация: "This book examines the application of artificial intelligence in machine learning, data mining in unstructured data sets or databases, web mining, and information retrieval"--
Доп.точки доступа:
Thomas, J. Joshua, (1973-) \editor.\
28.
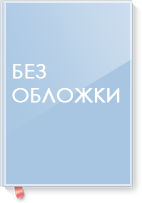
Подробнее
DDC 005.7
A 22
Advanced deep learning applications in big data analytics / / Hadj Ahmed Bouarara. - Hershey, PA : : Engineering Science Reference, an imprint of IGI Global,, [2021]. - 1 online resource. - (Advances in data mining and database management (ADMDM) book series). - Includes bibliographical references and index. - URL: https://library.dvfu.ru/lib/document/SK_ELIB/D71A6EE5-41D1-4E43-ADBB-209C649D2B2B. - ISBN 1799827933 (electronic book). - ISBN 9781799827931 (electronic bk.)
Description based on online resource; title from digital title page (viewed on December 07, 2020).
Параллельные издания: Print version: : Advanced deep learning applications in big data analytics. - Hershey, PA : Engineering Science Reference, an imprint of IGI Global, [2020]. - ISBN 9781799827917
Содержание:
Chapter 1. Advanced deep learning applications in big data analytics: introduction of Internet of things -- Chapter 2. Big data and cloud computing: a technological and literary background -- Chapter 3. Challenges and applications of data analytics in social perspectives: introduction of data science -- Chapter 4. Deep learning for social media text analytics -- Chapter 5. Deeplearning for computer vision problems: litterature review -- Chapter 6. Introduction of big data with analytics of big data -- Chapter 7. Machine learning in sentiment analysis over twitter: synthesis and comparative study -- Chapter 8. Machine learning techniques in spam detection -- Chapter 9. On swarm intelligence and its integration with Internet of things: challenges and applications -- Chapter 10. Physical characteristics of type 1 and 2 diabetic subjects: NCR, Indian-based computational perspective -- Chapter 11. Research information: bio-inspiration in information retrieval -- Chapter 12. Study on South Asian diabetic subjects on different attributes: a statistical approach.
~РУБ DDC 005.7
Рубрики: Big data.
Artificial intelligence.
Machine learning.
Artificial intelligence.
Big data.
Machine learning.
Аннотация: "This book explores the developing and application of deep learning in big data"--
Доп.точки доступа:
Bouarara, Hadj Ahmed, (1990-) \editor.\
A 22
Advanced deep learning applications in big data analytics / / Hadj Ahmed Bouarara. - Hershey, PA : : Engineering Science Reference, an imprint of IGI Global,, [2021]. - 1 online resource. - (Advances in data mining and database management (ADMDM) book series). - Includes bibliographical references and index. - URL: https://library.dvfu.ru/lib/document/SK_ELIB/D71A6EE5-41D1-4E43-ADBB-209C649D2B2B. - ISBN 1799827933 (electronic book). - ISBN 9781799827931 (electronic bk.)
Description based on online resource; title from digital title page (viewed on December 07, 2020).
Параллельные издания: Print version: : Advanced deep learning applications in big data analytics. - Hershey, PA : Engineering Science Reference, an imprint of IGI Global, [2020]. - ISBN 9781799827917
Содержание:
Chapter 1. Advanced deep learning applications in big data analytics: introduction of Internet of things -- Chapter 2. Big data and cloud computing: a technological and literary background -- Chapter 3. Challenges and applications of data analytics in social perspectives: introduction of data science -- Chapter 4. Deep learning for social media text analytics -- Chapter 5. Deeplearning for computer vision problems: litterature review -- Chapter 6. Introduction of big data with analytics of big data -- Chapter 7. Machine learning in sentiment analysis over twitter: synthesis and comparative study -- Chapter 8. Machine learning techniques in spam detection -- Chapter 9. On swarm intelligence and its integration with Internet of things: challenges and applications -- Chapter 10. Physical characteristics of type 1 and 2 diabetic subjects: NCR, Indian-based computational perspective -- Chapter 11. Research information: bio-inspiration in information retrieval -- Chapter 12. Study on South Asian diabetic subjects on different attributes: a statistical approach.
Рубрики: Big data.
Artificial intelligence.
Machine learning.
Artificial intelligence.
Big data.
Machine learning.
Аннотация: "This book explores the developing and application of deep learning in big data"--
Доп.точки доступа:
Bouarara, Hadj Ahmed, (1990-) \editor.\
29.
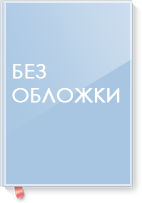
Подробнее
DDC 005.7
G 42
Ghavami, Peter K. ,
Big data management : data governance principles for big data analytics / Peter Ghavami. - Berlin ; Boston : Walter de Gruyter GmbH, ©2021. - 1 online resource (xviii, 155 pages) : il. - Includes bibliographical references and index. - URL: https://library.dvfu.ru/lib/document/SK_ELIB/48FF6C9F-DD59-4F1E-89B1-AC4BF9E33C56. - ISBN 9783110664065 (pdf). - ISBN 3110664062 (pdf). - ISBN 9783110664324 (epub). - ISBN 3110664321 (epub)
Print version record.
Параллельные издания: Print version: : Ghavami, Peter K. Big data management. - Berlin ; Boston : Walter de Gruyter GmbH, [2021]. - ISBN 9783110662917
Содержание:
Introduction -- 1. Introduction to Big Data -- 2. Enterprise Data Governance Directive -- 3. Data Risk Management -- 4. NoSQL Storage and Security Considerations -- 5. The Key Components of Big Data Governance -- 6. Big Data Governance Framework -- 7. Master Data Management -- 8. Big Data Governance Rules: Best Practices -- 9. Big Data Governance Best Practices -- 10. Big Data Governance Framework Program -- 11. Why Data and Model Risk Management? -- Summary.
~РУБ DDC 005.7
Рубрики: Big data.
Data mining.
BUSINESS & ECONOMICS / Government & Business
Big data
Data mining
Аннотация: Data analytics is core to business and decision making. The rapid increase in data volume, velocity and variety offers both opportunities and challenges. While open source solutions to store big data, like Hadoop, offer platforms for exploring value and insight from big data, they were not originally developed with data security and governance in mind. Big Data Management discusses numerous policies, strategies and recipes for managing big data. It addresses data security, privacy, controls and life cycle management offering modern principles and open source architectures for successful governance of big data. The author has collected best practices from the world's leading organizations that have successfully implemented big data platforms. The topics discussed cover the entire data management life cycle, data quality, data stewardship, regulatory considerations, data council, architectural and operational models are presented for successful management of big data. The book is a must-read for data scientists, data engineers and corporate leaders who are implementing big data platforms in their organizations
G 42
Ghavami, Peter K. ,
Big data management : data governance principles for big data analytics / Peter Ghavami. - Berlin ; Boston : Walter de Gruyter GmbH, ©2021. - 1 online resource (xviii, 155 pages) : il. - Includes bibliographical references and index. - URL: https://library.dvfu.ru/lib/document/SK_ELIB/48FF6C9F-DD59-4F1E-89B1-AC4BF9E33C56. - ISBN 9783110664065 (pdf). - ISBN 3110664062 (pdf). - ISBN 9783110664324 (epub). - ISBN 3110664321 (epub)
Print version record.
Параллельные издания: Print version: : Ghavami, Peter K. Big data management. - Berlin ; Boston : Walter de Gruyter GmbH, [2021]. - ISBN 9783110662917
Содержание:
Introduction -- 1. Introduction to Big Data -- 2. Enterprise Data Governance Directive -- 3. Data Risk Management -- 4. NoSQL Storage and Security Considerations -- 5. The Key Components of Big Data Governance -- 6. Big Data Governance Framework -- 7. Master Data Management -- 8. Big Data Governance Rules: Best Practices -- 9. Big Data Governance Best Practices -- 10. Big Data Governance Framework Program -- 11. Why Data and Model Risk Management? -- Summary.
Рубрики: Big data.
Data mining.
BUSINESS & ECONOMICS / Government & Business
Big data
Data mining
Аннотация: Data analytics is core to business and decision making. The rapid increase in data volume, velocity and variety offers both opportunities and challenges. While open source solutions to store big data, like Hadoop, offer platforms for exploring value and insight from big data, they were not originally developed with data security and governance in mind. Big Data Management discusses numerous policies, strategies and recipes for managing big data. It addresses data security, privacy, controls and life cycle management offering modern principles and open source architectures for successful governance of big data. The author has collected best practices from the world's leading organizations that have successfully implemented big data platforms. The topics discussed cover the entire data management life cycle, data quality, data stewardship, regulatory considerations, data council, architectural and operational models are presented for successful management of big data. The book is a must-read for data scientists, data engineers and corporate leaders who are implementing big data platforms in their organizations
30.
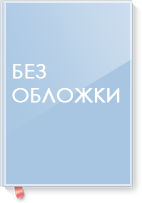
Подробнее
DDC 658.8/72
B 57
Big data analytics for improved accuracy, efficiency, and decision making in digital marketing / / Amandeep Singh, editor. - Hershey, PA : : Business Science Reference,, 2021. - 1 online resource. - Includes bibliographical references and index. - URL: https://library.dvfu.ru/lib/document/SK_ELIB/F2065C2A-9A4C-436B-9B27-F1AD60058960. - ISBN 9781799872337 (ebook). - ISBN 1799872335. - ISBN 9781799872344 (electronic bk.). - ISBN 1799872343 (electronic bk.)
Description based on print version record and CIP data provided by publisher; resource not viewed.
Параллельные издания: Print version: : Big data analytics for improved accuracy, efficiency, and decision making in digital marketing. - Hershey, PA : Business Science Reference, 2021. - ISBN 9781799872313
~РУБ DDC 658.8/72
Рубрики: Internet marketing--Statistical methods.
Decision making--Statistical methods.
Big data.
Аннотация: "This book will help the digital marketers to understand the Big Data in a better way and to get a meaning full information, which, will help the marketers to better market their products to the consumers"--
Доп.точки доступа:
Singh, Amandeep, (1982-) \editor.\
B 57
Big data analytics for improved accuracy, efficiency, and decision making in digital marketing / / Amandeep Singh, editor. - Hershey, PA : : Business Science Reference,, 2021. - 1 online resource. - Includes bibliographical references and index. - URL: https://library.dvfu.ru/lib/document/SK_ELIB/F2065C2A-9A4C-436B-9B27-F1AD60058960. - ISBN 9781799872337 (ebook). - ISBN 1799872335. - ISBN 9781799872344 (electronic bk.). - ISBN 1799872343 (electronic bk.)
Description based on print version record and CIP data provided by publisher; resource not viewed.
Параллельные издания: Print version: : Big data analytics for improved accuracy, efficiency, and decision making in digital marketing. - Hershey, PA : Business Science Reference, 2021. - ISBN 9781799872313
Рубрики: Internet marketing--Statistical methods.
Decision making--Statistical methods.
Big data.
Аннотация: "This book will help the digital marketers to understand the Big Data in a better way and to get a meaning full information, which, will help the marketers to better market their products to the consumers"--
Доп.точки доступа:
Singh, Amandeep, (1982-) \editor.\
Page 3, Results: 38