el cat en
База данных: ELS EBSCO eBook
Page 4, Results: 38
Отмеченные записи: 0
31.
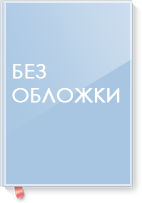
Подробнее
DDC 330.072/7
A 16
Abraham, Katharine G.
Big Data for Twenty-First-Century Economic Statistics [[electronic resource].] / Katharine G. Abraham, Jarmin, Ron S., Moyer, Brian C., Shapiro, Matthew D. - Chicago : : University of Chicago Press,, 2022. - 1 online resource (502 p.). - (National Bureau of Economic Research Studies in Income and Wealth ; ; v.79). - URL: https://library.dvfu.ru/lib/document/SK_ELIB/E054B194-D671-4C05-8A76-3E58C0CD4CD1. - ISBN 022680139X. - ISBN 9780226801391 (electronic bk.)
Description based upon print version of record.
Параллельные издания: Print version: : Abraham, Katharine G. Big Data for Twenty-First-Century Economic Statistics. - Chicago : University of Chicago Press,c2022. - ISBN 9780226801254
~РУБ DDC 330.072/7
Рубрики: Big data.
Economics--Statistical methods--Data processing.
Données volumineuses.
Économie politique--Méthodes statistiques--Informatique.
BUSINESS & ECONOMICS / General.
Аннотация: The papers in this volume analyze the deployment of Big Data to solve both existing and novel challenges in economic measurement. The existing infrastructure for the production of key economic statistics relies heavily on data collected through sample surveys and periodic censuses, together with administrative records generated in connection with tax administration. The increasing difficulty of obtaining survey and census responses threatens the viability of existing data collection approaches. The growing availability of new sources of Big Data--such as scanner data on purchases, credit card transaction records, payroll information, and prices of various goods scraped from the websites of online sellers--has changed the data landscape. These new sources of data hold the promise of allowing the statistical agencies to produce more accurate, more disaggregated, and more timely economic data to meet the needs of policymakers and other data users. This volume documents progress made toward that goal and the challenges to be overcome to realize the full potential of Big Data in the production of economic statistics. It describes the deployment of Big Data to solve both existing and novel challenges in economic measurement, and it will be of interest to statistical agency staff, academic researchers, and serious users of economic statistics.
Доп.точки доступа:
Jarmin, Ron S.
Moyer, Brian C.
Shapiro, Matthew D.
A 16
Abraham, Katharine G.
Big Data for Twenty-First-Century Economic Statistics [[electronic resource].] / Katharine G. Abraham, Jarmin, Ron S., Moyer, Brian C., Shapiro, Matthew D. - Chicago : : University of Chicago Press,, 2022. - 1 online resource (502 p.). - (National Bureau of Economic Research Studies in Income and Wealth ; ; v.79). - URL: https://library.dvfu.ru/lib/document/SK_ELIB/E054B194-D671-4C05-8A76-3E58C0CD4CD1. - ISBN 022680139X. - ISBN 9780226801391 (electronic bk.)
Description based upon print version of record.
Параллельные издания: Print version: : Abraham, Katharine G. Big Data for Twenty-First-Century Economic Statistics. - Chicago : University of Chicago Press,c2022. - ISBN 9780226801254
Рубрики: Big data.
Economics--Statistical methods--Data processing.
Données volumineuses.
Économie politique--Méthodes statistiques--Informatique.
BUSINESS & ECONOMICS / General.
Аннотация: The papers in this volume analyze the deployment of Big Data to solve both existing and novel challenges in economic measurement. The existing infrastructure for the production of key economic statistics relies heavily on data collected through sample surveys and periodic censuses, together with administrative records generated in connection with tax administration. The increasing difficulty of obtaining survey and census responses threatens the viability of existing data collection approaches. The growing availability of new sources of Big Data--such as scanner data on purchases, credit card transaction records, payroll information, and prices of various goods scraped from the websites of online sellers--has changed the data landscape. These new sources of data hold the promise of allowing the statistical agencies to produce more accurate, more disaggregated, and more timely economic data to meet the needs of policymakers and other data users. This volume documents progress made toward that goal and the challenges to be overcome to realize the full potential of Big Data in the production of economic statistics. It describes the deployment of Big Data to solve both existing and novel challenges in economic measurement, and it will be of interest to statistical agency staff, academic researchers, and serious users of economic statistics.
Доп.точки доступа:
Jarmin, Ron S.
Moyer, Brian C.
Shapiro, Matthew D.
32.
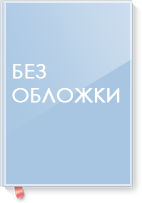
Подробнее
DDC 004.6782
E 11
Eagar, Gareth,.
Data engineering with AWS : : build and implement complex data pipelines using AWS / / Gareth Eagar. - Birmingham : : Packt Publishing,, 2021. - 1 online resource. - URL: https://library.dvfu.ru/lib/document/SK_ELIB/044794E3-9AD6-4626-8BE2-0248DCDD1FEB. - ISBN 1800569041. - ISBN 9781800569041 (electronic bk.)
Description based on CIP data; resource not viewed.
Параллельные издания: Print version: :
~РУБ DDC 004.6782
Рубрики: Cloud computing.
Big data.
Infonuagique.
Données volumineuses.
Big data.
Cloud computing.
E 11
Eagar, Gareth,.
Data engineering with AWS : : build and implement complex data pipelines using AWS / / Gareth Eagar. - Birmingham : : Packt Publishing,, 2021. - 1 online resource. - URL: https://library.dvfu.ru/lib/document/SK_ELIB/044794E3-9AD6-4626-8BE2-0248DCDD1FEB. - ISBN 1800569041. - ISBN 9781800569041 (electronic bk.)
Description based on CIP data; resource not viewed.
Параллельные издания: Print version: :
Рубрики: Cloud computing.
Big data.
Infonuagique.
Données volumineuses.
Big data.
Cloud computing.
33.
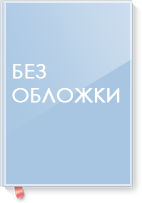
Подробнее
DDC 658/.0557
H 22
Handbook of research on foundations and applications of intelligent business analytics / / Zhaohao Sun, Zhiyou Wu. - Hershey, PA : : Business Science Reference, an imprint of IGI Global,, [2022]. - 1 online resource : : il. - (Advances in business information systems and analytics (ABISA) book series). - Includes bibliographical references and index. - URL: https://library.dvfu.ru/lib/document/SK_ELIB/70ADFCAD-460E-4E70-8D3F-2744DD2EB16C. - ISBN 9781799890195 (electronic book). - ISBN 1799890198 (electronic book). - ISBN 179989018X (electronic book). - ISBN 9781799890188 (electronic bk.)
Description based on online resource; title from digital title page (viewed on March 01, 2022).
Параллельные издания: Print version: : Handbook of research on foundations and applications of intelligent business analytics. - Hershey, PA : Business Science Reference, [2022]. - ISBN 9781799890164
Содержание:
Section 1. Foundations of intelligent business analytics. Chapter 1. The elements of intelligent business analytics: principles, techniques, and tools ; Chapter 2. Intelligent business analytics: need, functioning, challenges, and implications ; Chapter 3. Principles of a hybrid intelligence framework for augmented analytics ; Chapter 4. Designing business analytics projects (BAP): a five-step dashboarding cycle -- Section 2. Technologies for intelligent business analytics. Chapter 5. A process-oriented framework for regulating artificial intelligence systems ; Chapter 6. A Survey on hybrid case-based reasoning and deep learning systems for medical data classification ; Chapter 7. Big data analytics for search engine optimization in intelligent business ; Chapter 8. Machine learning and deep learning as new tools for business analytics ; Chapter 9. Semantic image analysis on social networks and data processing: review and future directions -- Section 3. Applications of intelligent business analytics. Chapter 10. Applying intelligent big data analytics in a smart airport business: value, adoption, and challenges ; Chapter 11. Immunize your organization: a people analytics exploration of the change in employee priorities after the COVID-19 lockdown ; Chapter 12. Undergraduate computer science capstone projects: experiences and examples in data science ; Chapter 13. The relationships between users' negative tweets, topic choices, and subjective well-being in Japan ; Chapter 14. A comparison of deep learning models in time series forecasting of web traffic data from Kaggle ; Chapter 15. Weibo analysis on Chinese cultural knowledge for gaming ; Chapter 16. Big data analytics and the discovery of the hidden data treasure from savings banks in Germany.
~РУБ DDC 658/.0557
Рубрики: Management--Data processing.
Big data.
Business planning--Statistical methods.
Big data.
Management--Data processing.
Аннотация: "This book addresses research issues by investigating into foundations, technologies, and applications of intelligent business analytics, offering theoretical foundations, technologies, methodologies, and applications of intelligent business analytics in an integrated way"--
Доп.точки доступа:
Sun, Zhaohao, \editor.\
Wu, Zhiyou, (1967-) \editor.\
H 22
Handbook of research on foundations and applications of intelligent business analytics / / Zhaohao Sun, Zhiyou Wu. - Hershey, PA : : Business Science Reference, an imprint of IGI Global,, [2022]. - 1 online resource : : il. - (Advances in business information systems and analytics (ABISA) book series). - Includes bibliographical references and index. - URL: https://library.dvfu.ru/lib/document/SK_ELIB/70ADFCAD-460E-4E70-8D3F-2744DD2EB16C. - ISBN 9781799890195 (electronic book). - ISBN 1799890198 (electronic book). - ISBN 179989018X (electronic book). - ISBN 9781799890188 (electronic bk.)
Description based on online resource; title from digital title page (viewed on March 01, 2022).
Параллельные издания: Print version: : Handbook of research on foundations and applications of intelligent business analytics. - Hershey, PA : Business Science Reference, [2022]. - ISBN 9781799890164
Содержание:
Section 1. Foundations of intelligent business analytics. Chapter 1. The elements of intelligent business analytics: principles, techniques, and tools ; Chapter 2. Intelligent business analytics: need, functioning, challenges, and implications ; Chapter 3. Principles of a hybrid intelligence framework for augmented analytics ; Chapter 4. Designing business analytics projects (BAP): a five-step dashboarding cycle -- Section 2. Technologies for intelligent business analytics. Chapter 5. A process-oriented framework for regulating artificial intelligence systems ; Chapter 6. A Survey on hybrid case-based reasoning and deep learning systems for medical data classification ; Chapter 7. Big data analytics for search engine optimization in intelligent business ; Chapter 8. Machine learning and deep learning as new tools for business analytics ; Chapter 9. Semantic image analysis on social networks and data processing: review and future directions -- Section 3. Applications of intelligent business analytics. Chapter 10. Applying intelligent big data analytics in a smart airport business: value, adoption, and challenges ; Chapter 11. Immunize your organization: a people analytics exploration of the change in employee priorities after the COVID-19 lockdown ; Chapter 12. Undergraduate computer science capstone projects: experiences and examples in data science ; Chapter 13. The relationships between users' negative tweets, topic choices, and subjective well-being in Japan ; Chapter 14. A comparison of deep learning models in time series forecasting of web traffic data from Kaggle ; Chapter 15. Weibo analysis on Chinese cultural knowledge for gaming ; Chapter 16. Big data analytics and the discovery of the hidden data treasure from savings banks in Germany.
Рубрики: Management--Data processing.
Big data.
Business planning--Statistical methods.
Big data.
Management--Data processing.
Аннотация: "This book addresses research issues by investigating into foundations, technologies, and applications of intelligent business analytics, offering theoretical foundations, technologies, methodologies, and applications of intelligent business analytics in an integrated way"--
Доп.точки доступа:
Sun, Zhaohao, \editor.\
Wu, Zhiyou, (1967-) \editor.\
34.
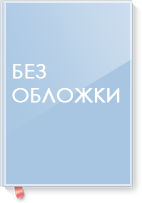
Подробнее
DDC 005.7
W 65
Widjaja, Adi,.
Data engineering with Google Cloud Platform : : a practical guide to operationalizing scalable data analytics systems on GCP / / Adi Widjaja. - Birmingham : : Packt Publishing,, 2022. - 1 online resource. - URL: https://library.dvfu.ru/lib/document/SK_ELIB/8F1FC074-4A68-497E-94E4-63F8B79DCB25. - ISBN 1800565062. - ISBN 9781800565067 (electronic bk.)
Print on demand edition. Description based on CIP data; resource not viewed.
Параллельные издания: Print version: :
~РУБ DDC 005.7
Рубрики: Big data.
Cloud computing.
Web services.
Computer organization.
Données volumineuses.
Infonuagique.
Services Web.
Ordinateurs--Conception et construction.
Big data.
Cloud computing.
Computer organization.
Web services.
Аннотация: Build and deploy your own data pipelines on GCP, make key architectural decisions, and gain the confidence to boost your career as a data engineer Key Features Understand data engineering concepts, the role of a data engineer, and the benefits of using GCP for building your solution Learn how to use the various GCP products to ingest, consume, and transform data and orchestrate pipelines Discover tips to prepare for and pass the Professional Data Engineer exam Book Description With this book, you'll understand how the highly scalable Google Cloud Platform (GCP) enables data engineers to create end-to-end data pipelines right from storing and processing data and workflow orchestration to presenting data through visualization dashboards. Starting with a quick overview of the fundamental concepts of data engineering, you'll learn the various responsibilities of a data engineer and how GCP plays a vital role in fulfilling those responsibilities. As you progress through the chapters, you'll be able to leverage GCP products to build a sample data warehouse using Cloud Storage and BigQuery and a data lake using Dataproc. The book gradually takes you through operations such as data ingestion, data cleansing, transformation, and integrating data with other sources. You'll learn how to design IAM for data governance, deploy ML pipelines with the Vertex AI, leverage pre-built GCP models as a service, and visualize data with Google Data Studio to build compelling reports. Finally, you'll find tips on how to boost your career as a data engineer, take the Professional Data Engineer certification exam, and get ready to become an expert in data engineering with GCP. By the end of this data engineering book, you'll have developed the skills to perform core data engineering tasks and build efficient ETL data pipelines with GCP. What you will learn Load data into BigQuery and materialize its output for downstream consumption Build data pipeline orchestration using Cloud Composer Develop Airflow jobs to orchestrate and automate a data warehouse Build a Hadoop data lake, create ephemeral clusters, and run jobs on the Dataproc cluster Leverage Pub/Sub for messaging and ingestion for event-driven systems Use Dataflow to perform ETL on streaming data Unlock the power of your data with Data Studio Calculate the GCP cost estimation for your end-to-end data solutions Who this book is for This book is for data engineers, data analysts, and anyone looking to design and manage data processing pipelines using GCP. You'll find this book useful if you are preparing to take Google's Professional Data Engineer exam. Beginner-level understanding of data science, the Python programming language, and Linux commands is necessary. A basic understanding of data processing and cloud computing, in general, will help you make the most out of this book.
W 65
Widjaja, Adi,.
Data engineering with Google Cloud Platform : : a practical guide to operationalizing scalable data analytics systems on GCP / / Adi Widjaja. - Birmingham : : Packt Publishing,, 2022. - 1 online resource. - URL: https://library.dvfu.ru/lib/document/SK_ELIB/8F1FC074-4A68-497E-94E4-63F8B79DCB25. - ISBN 1800565062. - ISBN 9781800565067 (electronic bk.)
Print on demand edition. Description based on CIP data; resource not viewed.
Параллельные издания: Print version: :
Рубрики: Big data.
Cloud computing.
Web services.
Computer organization.
Données volumineuses.
Infonuagique.
Services Web.
Ordinateurs--Conception et construction.
Big data.
Cloud computing.
Computer organization.
Web services.
Аннотация: Build and deploy your own data pipelines on GCP, make key architectural decisions, and gain the confidence to boost your career as a data engineer Key Features Understand data engineering concepts, the role of a data engineer, and the benefits of using GCP for building your solution Learn how to use the various GCP products to ingest, consume, and transform data and orchestrate pipelines Discover tips to prepare for and pass the Professional Data Engineer exam Book Description With this book, you'll understand how the highly scalable Google Cloud Platform (GCP) enables data engineers to create end-to-end data pipelines right from storing and processing data and workflow orchestration to presenting data through visualization dashboards. Starting with a quick overview of the fundamental concepts of data engineering, you'll learn the various responsibilities of a data engineer and how GCP plays a vital role in fulfilling those responsibilities. As you progress through the chapters, you'll be able to leverage GCP products to build a sample data warehouse using Cloud Storage and BigQuery and a data lake using Dataproc. The book gradually takes you through operations such as data ingestion, data cleansing, transformation, and integrating data with other sources. You'll learn how to design IAM for data governance, deploy ML pipelines with the Vertex AI, leverage pre-built GCP models as a service, and visualize data with Google Data Studio to build compelling reports. Finally, you'll find tips on how to boost your career as a data engineer, take the Professional Data Engineer certification exam, and get ready to become an expert in data engineering with GCP. By the end of this data engineering book, you'll have developed the skills to perform core data engineering tasks and build efficient ETL data pipelines with GCP. What you will learn Load data into BigQuery and materialize its output for downstream consumption Build data pipeline orchestration using Cloud Composer Develop Airflow jobs to orchestrate and automate a data warehouse Build a Hadoop data lake, create ephemeral clusters, and run jobs on the Dataproc cluster Leverage Pub/Sub for messaging and ingestion for event-driven systems Use Dataflow to perform ETL on streaming data Unlock the power of your data with Data Studio Calculate the GCP cost estimation for your end-to-end data solutions Who this book is for This book is for data engineers, data analysts, and anyone looking to design and manage data processing pipelines using GCP. You'll find this book useful if you are preparing to take Google's Professional Data Engineer exam. Beginner-level understanding of data science, the Python programming language, and Linux commands is necessary. A basic understanding of data processing and cloud computing, in general, will help you make the most out of this book.
35.
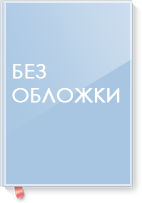
Подробнее
DDC 005.7
N 73
Noise filtering for big data analytics / / Souvik Bhattacharyya, Koushik Ghosh (eds.). - Berlin ; ; Boston: : De Gruyter,, [2022]. - 1 online resource : : il. - (De Gruyter series on the applications of mathematics in engineering and information sciences ; ; volume 12). - Includes bibliographical references and index. - URL: https://library.dvfu.ru/lib/document/SK_ELIB/A13D0852-2871-4AC1-8532-78CA054C6941. - ISBN 9783110697216 (electronic book). - ISBN 3110697211 (electronic book). - ISBN 9783110697261 (electronic publication). - ISBN 3110697262 (electronic publication)
Description based on online resource; title from digital title page (viewed on July 29, 2022).
Параллельные издания: Print version: : Noise filtering for big data analytics. - Berlin : De Gruyter, 2022. - ISBN 9783110697094
~РУБ DDC 005.7
Рубрики: Big data.
Data mining.
Information filtering systems.
Angewandte Mathematik.
Big Data.
Künstliche Intelligenz.
Maschinelles Lernen.
COMPUTERS / Information Technology.
Аннотация: This book explains how to perform data de-noising, in large scale, with a satisfactory level of accuracy. Three main issues are considered. Firstly, how to eliminate the error propagation from one stage to next stages while developing a filtered model. Secondly, how to maintain the positional importance of data whilst purifying it. Finally, preservation of memory in the data is crucial to extract smart data from noisy big data. If, after the application of any form of smoothing or filtering, the memory of the corresponding data changes heavily, then the final data may lose some important information. This may lead to wrong or erroneous conclusions. But, when anticipating any loss of information due to smoothing or filtering, one cannot avoid the process of denoising as on the other hand any kind of analysis of big data in the presence of noise can be misleading. So, the entire process demands very careful execution with efficient and smart models in order to effectively deal with it.
Доп.точки доступа:
Bhattacharyya, Souvik, \editor.\
Ghosh, Koushik, \editor.\
N 73
Noise filtering for big data analytics / / Souvik Bhattacharyya, Koushik Ghosh (eds.). - Berlin ; ; Boston: : De Gruyter,, [2022]. - 1 online resource : : il. - (De Gruyter series on the applications of mathematics in engineering and information sciences ; ; volume 12). - Includes bibliographical references and index. - URL: https://library.dvfu.ru/lib/document/SK_ELIB/A13D0852-2871-4AC1-8532-78CA054C6941. - ISBN 9783110697216 (electronic book). - ISBN 3110697211 (electronic book). - ISBN 9783110697261 (electronic publication). - ISBN 3110697262 (electronic publication)
Description based on online resource; title from digital title page (viewed on July 29, 2022).
Параллельные издания: Print version: : Noise filtering for big data analytics. - Berlin : De Gruyter, 2022. - ISBN 9783110697094
Рубрики: Big data.
Data mining.
Information filtering systems.
Angewandte Mathematik.
Big Data.
Künstliche Intelligenz.
Maschinelles Lernen.
COMPUTERS / Information Technology.
Аннотация: This book explains how to perform data de-noising, in large scale, with a satisfactory level of accuracy. Three main issues are considered. Firstly, how to eliminate the error propagation from one stage to next stages while developing a filtered model. Secondly, how to maintain the positional importance of data whilst purifying it. Finally, preservation of memory in the data is crucial to extract smart data from noisy big data. If, after the application of any form of smoothing or filtering, the memory of the corresponding data changes heavily, then the final data may lose some important information. This may lead to wrong or erroneous conclusions. But, when anticipating any loss of information due to smoothing or filtering, one cannot avoid the process of denoising as on the other hand any kind of analysis of big data in the presence of noise can be misleading. So, the entire process demands very careful execution with efficient and smart models in order to effectively deal with it.
Доп.точки доступа:
Bhattacharyya, Souvik, \editor.\
Ghosh, Koushik, \editor.\
36.
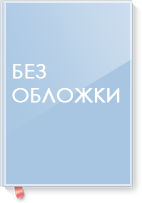
Подробнее
DDC 658.8/72
B 57
Big Data : : A Road Map for Successful Digital Marketing / / edited by Amandeep Singh, Rohit Bansal and Sandhir Sharma. - 1515/9783110733716. - Berlin ; ; Boston : : De Gruyter,, ©2022. - 1 online resource (x, 244 pages) : il ( час. мин.), 1515/9783110733716. - In English. - Includes index and bibliographical references. - URL: https://library.dvfu.ru/lib/document/SK_ELIB/76F83248-6340-41C1-9825-37531BCCDCAD. - ISBN 3110733714 (electronic book). - ISBN 9783110733716 (electronic bk.)
Description based on online resource; title from PDF title page (publisher's Web site, viewed 30. Aug 2022).
Параллельные издания:
1. Print version: :
2. Print version: :
~РУБ DDC 658.8/72
Рубрики: Internet marketing--Technological innovations.
Big data.
BUSINESS & ECONOMICS / Marketing / General.
Big data.
Кл.слова (ненормированные):
Role of Big Data, Digital Marketing, Big Data Information, Data Analytics, Business Information System, New Age Marketing, New Age Marketing.
Аннотация: Imagine being able to target an audience made up of highly qualified and purchase-ready prospects and easily building them into loyal clients by anticipating their needs and hence offering true value. This is the power of big data for digital marketing. Big Data: A Roadmap for Successful Digital Marketing explores recent trends in the use of big data to predict consumer behavior, strategies to engage online customers, integration of big data with other data sources, and its applications in social media analytics, mobile marketing, search engine optimization and customer relationship management. As the marketing world moves into a data-focused future, the success of marketing efforts will be wholly based on attention to detail in data analysis and effectively acting on insights in order to implement changes that will deliver improved results. This book will help professionals succeed in their digital marketing efforts as well as provide food for thought for students and researchers in the fields of digital marketing, customer behavior and big data analytics.
Доп.точки доступа:
Singh, Amandeep, (1982-) \editor.\
Bansal, Rohit, (1982-) \editor.\
Sharma, Sandhir, \editor,.\
Abadia, Rhodora,
Aggarwal, Kriti,
Ahmad, Ashfaq,
Balamurugan, S.,
Baral, Manish Mohan,
Cervantes Flores, Oliver Moisés,
Cervantes, Valeria Meléndez,
Cervera, Claudia Malćn,
Chimhundu, Ranga,
De la Vega Meneses, José Gerardo,
Dhaya, R.,
Goyal, Gulshan,
Gupta, Alock,
Hassan, Rumman,
Jana, Bhaswati,
Kalyani, B. J. D.,
Kanthavel, R.,
Kapoor, Komal,
Kaur, Harmanpreet,
Kaushik, Tanvi,
Khoi, Bui Huy,
Krishan, Ram,
Malhotra, Rohit,
Malik, Reena,
Mittal, Vipin,
Mukherjee, Subhodeep,
Palanivelrajan, M.,
Putri, Seprianti Eka,
Rabby, Fazla,
Rana, Sudhir,
Rasul, Tareq,
Sandhu, Namrata,
Selvalakshmi, M.,
Singh, Dilpreet,
Trivedi, Sonal,
Venkataiah, Chittipaka,
B 57
Big Data : : A Road Map for Successful Digital Marketing / / edited by Amandeep Singh, Rohit Bansal and Sandhir Sharma. - 1515/9783110733716. - Berlin ; ; Boston : : De Gruyter,, ©2022. - 1 online resource (x, 244 pages) : il ( час. мин.), 1515/9783110733716. - In English. - Includes index and bibliographical references. - URL: https://library.dvfu.ru/lib/document/SK_ELIB/76F83248-6340-41C1-9825-37531BCCDCAD. - ISBN 3110733714 (electronic book). - ISBN 9783110733716 (electronic bk.)
Description based on online resource; title from PDF title page (publisher's Web site, viewed 30. Aug 2022).
Параллельные издания:
1. Print version: :
2. Print version: :
Рубрики: Internet marketing--Technological innovations.
Big data.
BUSINESS & ECONOMICS / Marketing / General.
Big data.
Кл.слова (ненормированные):
Role of Big Data, Digital Marketing, Big Data Information, Data Analytics, Business Information System, New Age Marketing, New Age Marketing.
Аннотация: Imagine being able to target an audience made up of highly qualified and purchase-ready prospects and easily building them into loyal clients by anticipating their needs and hence offering true value. This is the power of big data for digital marketing. Big Data: A Roadmap for Successful Digital Marketing explores recent trends in the use of big data to predict consumer behavior, strategies to engage online customers, integration of big data with other data sources, and its applications in social media analytics, mobile marketing, search engine optimization and customer relationship management. As the marketing world moves into a data-focused future, the success of marketing efforts will be wholly based on attention to detail in data analysis and effectively acting on insights in order to implement changes that will deliver improved results. This book will help professionals succeed in their digital marketing efforts as well as provide food for thought for students and researchers in the fields of digital marketing, customer behavior and big data analytics.
Доп.точки доступа:
Singh, Amandeep, (1982-) \editor.\
Bansal, Rohit, (1982-) \editor.\
Sharma, Sandhir, \editor,.\
Abadia, Rhodora,
Aggarwal, Kriti,
Ahmad, Ashfaq,
Balamurugan, S.,
Baral, Manish Mohan,
Cervantes Flores, Oliver Moisés,
Cervantes, Valeria Meléndez,
Cervera, Claudia Malćn,
Chimhundu, Ranga,
De la Vega Meneses, José Gerardo,
Dhaya, R.,
Goyal, Gulshan,
Gupta, Alock,
Hassan, Rumman,
Jana, Bhaswati,
Kalyani, B. J. D.,
Kanthavel, R.,
Kapoor, Komal,
Kaur, Harmanpreet,
Kaushik, Tanvi,
Khoi, Bui Huy,
Krishan, Ram,
Malhotra, Rohit,
Malik, Reena,
Mittal, Vipin,
Mukherjee, Subhodeep,
Palanivelrajan, M.,
Putri, Seprianti Eka,
Rabby, Fazla,
Rana, Sudhir,
Rasul, Tareq,
Sandhu, Namrata,
Selvalakshmi, M.,
Singh, Dilpreet,
Trivedi, Sonal,
Venkataiah, Chittipaka,
37.
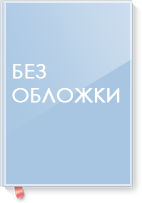
Подробнее
DDC 005.7
G 42
Ghavami, Peter,.
Big Data Analytics Methods : : Analytics Techniques in Data Mining, Deep Learning and Natural Language Processing / / Peter Ghavami. - 2nd Edition. - 1515/9781547401567. - Berlin ; ; Boston : : De Gruyter,, ©2020. - 1 online resource (XVI, 238 pages) : гербы ( час. мин.), 1515/9781547401567. - In English. - URL: https://library.dvfu.ru/lib/document/SK_ELIB/43121066-BC0F-4D45-885E-683DB62AC286. - ISBN 9781547401567. - ISBN 1547401567. - ISBN 9781547401581. - ISBN 1547401583. - ISBN 9781547417957. - ISBN 1547417951
Description based on online resource; title from PDF title page (publisher's Web site, viewed 21. Dez 2019).
Параллельные издания:
1.
2.
~РУБ DDC 005.7
Рубрики: Big data.
Data analysis.
Data mining.
Machine learning.
Neural networks.
BUSINESS & ECONOMICS / Information Management.
Big data.
Data mining.
Natural language processing (Computer science)
Big data.
Data mining.
Natural language processing (Computer science)
Аннотация: Big Data Analytics Methods unveils secrets to advanced analytics techniques ranging from machine learning, random forest classifiers, predictive modeling, cluster analysis, natural language processing (NLP), Kalman filtering and ensembles of models for optimal accuracy of analysis and prediction. More than 100 analytics techniques and methods provide big data professionals, business intelligence professionals and citizen data scientists insight on how to overcome challenges and avoid common pitfalls and traps in data analytics. The book offers solutions and tips on handling missing data, noisy and dirty data, error reduction and boosting signal to reduce noise. It discusses data visualization, prediction, optimization, artificial intelligence, regression analysis, the Cox hazard model and many analytics using case examples with applications in the healthcare, transportation, retail, telecommunication, consulting, manufacturing, energy and financial services industries. This book's state of the art treatment of advanced data analytics methods and important best practices will help readers succeed in data analytics.
G 42
Ghavami, Peter,.
Big Data Analytics Methods : : Analytics Techniques in Data Mining, Deep Learning and Natural Language Processing / / Peter Ghavami. - 2nd Edition. - 1515/9781547401567. - Berlin ; ; Boston : : De Gruyter,, ©2020. - 1 online resource (XVI, 238 pages) : гербы ( час. мин.), 1515/9781547401567. - In English. - URL: https://library.dvfu.ru/lib/document/SK_ELIB/43121066-BC0F-4D45-885E-683DB62AC286. - ISBN 9781547401567. - ISBN 1547401567. - ISBN 9781547401581. - ISBN 1547401583. - ISBN 9781547417957. - ISBN 1547417951
Description based on online resource; title from PDF title page (publisher's Web site, viewed 21. Dez 2019).
Параллельные издания:
1.
2.
Рубрики: Big data.
Data analysis.
Data mining.
Machine learning.
Neural networks.
BUSINESS & ECONOMICS / Information Management.
Big data.
Data mining.
Natural language processing (Computer science)
Big data.
Data mining.
Natural language processing (Computer science)
Аннотация: Big Data Analytics Methods unveils secrets to advanced analytics techniques ranging from machine learning, random forest classifiers, predictive modeling, cluster analysis, natural language processing (NLP), Kalman filtering and ensembles of models for optimal accuracy of analysis and prediction. More than 100 analytics techniques and methods provide big data professionals, business intelligence professionals and citizen data scientists insight on how to overcome challenges and avoid common pitfalls and traps in data analytics. The book offers solutions and tips on handling missing data, noisy and dirty data, error reduction and boosting signal to reduce noise. It discusses data visualization, prediction, optimization, artificial intelligence, regression analysis, the Cox hazard model and many analytics using case examples with applications in the healthcare, transportation, retail, telecommunication, consulting, manufacturing, energy and financial services industries. This book's state of the art treatment of advanced data analytics methods and important best practices will help readers succeed in data analytics.
38.
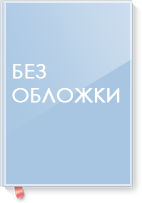
Подробнее
DDC 658.4038
S 34
Schmarzo, Bill.
The the Economics of Data, Analytics, and Digital Transformation : : The Theorems, Laws, and Empowerments to Guide Your Organization's Digital Transformation. / Bill. Schmarzo, Borne, Kirk. - Birmingham : : Packt Publishing, Limited,, 2020. - 1 online resource (253 p.). - URL: https://library.dvfu.ru/lib/document/SK_ELIB/E1647E56-5900-4D04-AD98-D25B2220AA9B. - ISBN 9781800569133 (electronic book). - ISBN 1800569130 (electronic book)
Параллельные издания: Print version: : Schmarzo, Bill The the Economics of Data, Analytics, and Digital Transformation : The Theorems, Laws, and Empowerments to Guide Your Organization's Digital Transformation. - Birmingham : Packt Publishing, Limited,c2020. - ISBN 9781800561410
Содержание:
Cover -- Copyright -- Packt Page -- Foreword -- Contributor -- Table of Content -- Preface -- Chapter 1: The CEO Mandate: Become Value-driven, Not Data-driven -- The Data- and Value-Driven Mindsets, Defined -- Understanding the Big Data Business Model Maturity Index Phases -- Navigating the Big Data Business Model Maturity Index -- Transitioning from Business Monitoring to Business Insights -- Transitioning from Business Insights to Business Optimization -- Transitioning from Business Optimization to Insights Monetization -- Transitioning from Insights Monetization to Digital Transformation
Testing the Big Data Business Model Maturity Index -- Summary -- Further Reading -- Homework -- Chapter 2: Value Engineering: The Secret Sauce for Data Science Success -- Step 1: Identify a Strategic Business Initiative -- Step 2: Identify Key Business Stakeholders -- Step 3: Brainstorm and Prioritize Decisions (Use Cases) -- Step 4: Identify Supporting Analytics -- Step 5: Identify Potential Data Sources and Instrumentation Strategy -- Step 6: Identify Supporting Architecture and Technologies -- Summary -- Homework -- Chapter 3: A Review of Basic Economic Concepts -- The Economic Value Curve
The Law of Supply and Demand -- The Economic Multiplier Effect -- Marginal Costs and Sunk Costs -- Scarcity -- Postponement Theory -- Efficiency -- Capital -- Price Elasticity -- The Economic Utility Function -- Summary -- Further Reading -- Homework -- Chapter 4: University of San Francisco Economic Value of Data Research Paper -- Introduction -- Creating the Collaborative Value Creation Framework -- Chipotle Use Case -- Summary -- Citations -- Chapter 5: The Economic Value of Data Theorems -- EvD Theorem #1: Data, By Itself, Provides Little Value
EvD Theorem #2: Predictions, Not Data, Drive Value -- EvD Theorem #3: Predictions Drive Value Through Use Cases -- EvD Theorem #4: The Data Economic Multiplier Effect is the Real Game-changer -- EvD Theorem #5: Predictions Enable ""Do More with Less -- The Economic Value of Data Calculation -- Summary -- Further Reading -- Homework -- Chapter 6: The Economics of Artificial Intelligence -- Orphaned Analytics -- Role of Analytic Modules -- Composable, Reusable, Continuously Learning Analytic Module Architecture -- A Quick Primer on Deep Learning, Reinforcement Learning, and Artificial Intelligence
Case Study #1: Google TensorFlow -- Case Study #2: Tesla Autonomous Vehicles -- The Autonomous Holy Grail of AI -- Summary -- Further Reading -- Homework -- Chapter 7: The Schmarzo Economic Digital Assest Valuation Theorem -- The Economies of Scale -- The Economies of Learning -- Digital Economics Effect #1: Marginal Costs Flatten -- Digital Economics Effect #2: Economic Value of Digital Assets Grows -- Digital Economics Effect #3: Economic Value of Digital Assets Accelerates -- Implementing the Schmarzo Economic Digital Asset Valuation Theorem -- Summary -- Further Reading -- Homework
~РУБ DDC 658.4038
Рубрики: Information technology--Management.
Big data.
Database design & theory.
Data capture & analysis.
Information visualization.
Information architecture.
Computers--Data Processing.
Computers--Data Modeling & Design.
Big data.
Information technology--Management.
Аннотация: A comprehensive guide for seasoned business leaders who struggle with where and how to exploit the economics of data and analytics to gain true value from data, accelerate company operations through AI, and guide their digital transformation.
Доп.точки доступа:
Borne, Kirk.
S 34
Schmarzo, Bill.
The the Economics of Data, Analytics, and Digital Transformation : : The Theorems, Laws, and Empowerments to Guide Your Organization's Digital Transformation. / Bill. Schmarzo, Borne, Kirk. - Birmingham : : Packt Publishing, Limited,, 2020. - 1 online resource (253 p.). - URL: https://library.dvfu.ru/lib/document/SK_ELIB/E1647E56-5900-4D04-AD98-D25B2220AA9B. - ISBN 9781800569133 (electronic book). - ISBN 1800569130 (electronic book)
Параллельные издания: Print version: : Schmarzo, Bill The the Economics of Data, Analytics, and Digital Transformation : The Theorems, Laws, and Empowerments to Guide Your Organization's Digital Transformation. - Birmingham : Packt Publishing, Limited,c2020. - ISBN 9781800561410
Содержание:
Cover -- Copyright -- Packt Page -- Foreword -- Contributor -- Table of Content -- Preface -- Chapter 1: The CEO Mandate: Become Value-driven, Not Data-driven -- The Data- and Value-Driven Mindsets, Defined -- Understanding the Big Data Business Model Maturity Index Phases -- Navigating the Big Data Business Model Maturity Index -- Transitioning from Business Monitoring to Business Insights -- Transitioning from Business Insights to Business Optimization -- Transitioning from Business Optimization to Insights Monetization -- Transitioning from Insights Monetization to Digital Transformation
Testing the Big Data Business Model Maturity Index -- Summary -- Further Reading -- Homework -- Chapter 2: Value Engineering: The Secret Sauce for Data Science Success -- Step 1: Identify a Strategic Business Initiative -- Step 2: Identify Key Business Stakeholders -- Step 3: Brainstorm and Prioritize Decisions (Use Cases) -- Step 4: Identify Supporting Analytics -- Step 5: Identify Potential Data Sources and Instrumentation Strategy -- Step 6: Identify Supporting Architecture and Technologies -- Summary -- Homework -- Chapter 3: A Review of Basic Economic Concepts -- The Economic Value Curve
The Law of Supply and Demand -- The Economic Multiplier Effect -- Marginal Costs and Sunk Costs -- Scarcity -- Postponement Theory -- Efficiency -- Capital -- Price Elasticity -- The Economic Utility Function -- Summary -- Further Reading -- Homework -- Chapter 4: University of San Francisco Economic Value of Data Research Paper -- Introduction -- Creating the Collaborative Value Creation Framework -- Chipotle Use Case -- Summary -- Citations -- Chapter 5: The Economic Value of Data Theorems -- EvD Theorem #1: Data, By Itself, Provides Little Value
EvD Theorem #2: Predictions, Not Data, Drive Value -- EvD Theorem #3: Predictions Drive Value Through Use Cases -- EvD Theorem #4: The Data Economic Multiplier Effect is the Real Game-changer -- EvD Theorem #5: Predictions Enable ""Do More with Less -- The Economic Value of Data Calculation -- Summary -- Further Reading -- Homework -- Chapter 6: The Economics of Artificial Intelligence -- Orphaned Analytics -- Role of Analytic Modules -- Composable, Reusable, Continuously Learning Analytic Module Architecture -- A Quick Primer on Deep Learning, Reinforcement Learning, and Artificial Intelligence
Case Study #1: Google TensorFlow -- Case Study #2: Tesla Autonomous Vehicles -- The Autonomous Holy Grail of AI -- Summary -- Further Reading -- Homework -- Chapter 7: The Schmarzo Economic Digital Assest Valuation Theorem -- The Economies of Scale -- The Economies of Learning -- Digital Economics Effect #1: Marginal Costs Flatten -- Digital Economics Effect #2: Economic Value of Digital Assets Grows -- Digital Economics Effect #3: Economic Value of Digital Assets Accelerates -- Implementing the Schmarzo Economic Digital Asset Valuation Theorem -- Summary -- Further Reading -- Homework
Рубрики: Information technology--Management.
Big data.
Database design & theory.
Data capture & analysis.
Information visualization.
Information architecture.
Computers--Data Processing.
Computers--Data Modeling & Design.
Big data.
Information technology--Management.
Аннотация: A comprehensive guide for seasoned business leaders who struggle with where and how to exploit the economics of data and analytics to gain true value from data, accelerate company operations through AI, and guide their digital transformation.
Доп.точки доступа:
Borne, Kirk.
Page 4, Results: 38