База данных: Электронная библиотека
Страница 1, Результатов: 3
Отмеченные записи: 0
1.
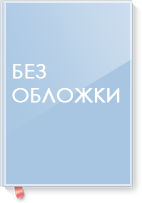
Подробнее
DDC 658.830285
B 64
Blanchard, Tommy,.
Data science for marketing analytics / / Tommy Blanchard, Debasish Behera, Pranshu Bhatnagar. - Birmingham, UK : : Packt Publishing,, 2019. - 1 online resource (1 volume) : : il. - Includes bibliographical references. - URL: https://library.dvfu.ru/lib/document/SK_ELIB/3DB5D77C-C251-4493-8806-F32E4AF8633A. - ISBN 9781789952100 (electronic bk.). - ISBN 1789952107 (electronic bk.)
Description based on online resource; title from copyright page (Safari, viewed May 15, 2019).
~РУБ DDC 658.830285
Рубрики: Marketing--Data processing.
Marketing research.
Python (Computer program language)
Information visualization.
BUSINESS & ECONOMICS / Industrial Management
BUSINESS & ECONOMICS / Management
BUSINESS & ECONOMICS / Management Science
BUSINESS & ECONOMICS / Organizational Behavior
Аннотация: Explore new and more sophisticated tools that reduce your marketing analytics efforts and give you precise results Key Features Study new techniques for marketing analytics Explore uses of machine learning to power your marketing analyses Work through each stage of data analytics with the help of multiple examples and exercises Book Description Data Science for Marketing Analytics covers every stage of data analytics, from working with a raw dataset to segmenting a population and modeling different parts of the population based on the segments. The book starts by teaching you how to use Python libraries, such as pandas and Matplotlib, to read data from Python, manipulate it, and create plots, using both categorical and continuous variables. Then, you'll learn how to segment a population into groups and use different clustering techniques to evaluate customer segmentation. As you make your way through the chapters, you'll explore ways to evaluate and select the best segmentation approach, and go on to create a linear regression model on customer value data to predict lifetime value. In the concluding chapters, you'll gain an understanding of regression techniques and tools for evaluating regression models, and explore ways to predict customer choice using classification algorithms. Finally, you'll apply these techniques to create a churn model for modeling customer product choices. By the end of this book, you will be able to build your own marketing reporting and interactive dashboard solutions. What you will learn Analyze and visualize data in Python using pandas and Matplotlib Study clustering techniques, such as hierarchical and k-means clustering Create customer segments based on manipulated data Predict customer lifetime value using linear regression Use classification algorithms to understand customer choice Optimize classification algorithms to extract maximal information Who this book is for Data Science for Marketing Analytics is designed for developers and marketing analysts looking to use new, more sophisticated tools in their marketing analytics efforts. It'll help if you have prior experience of coding in Python and knowledge of high school level mathematics. Some experience with databases, Excel, statistics, or Tableau is useful but not necessary. Downloading the example code for this book You can download the example code files for all Packt books you have purchased from your account at http://www.PacktPub.com. If you purchased ...
Доп.точки доступа:
Bhatnagar, Pranshu, \author.\
Behera, Debasish, \author.\
B 64
Blanchard, Tommy,.
Data science for marketing analytics / / Tommy Blanchard, Debasish Behera, Pranshu Bhatnagar. - Birmingham, UK : : Packt Publishing,, 2019. - 1 online resource (1 volume) : : il. - Includes bibliographical references. - URL: https://library.dvfu.ru/lib/document/SK_ELIB/3DB5D77C-C251-4493-8806-F32E4AF8633A. - ISBN 9781789952100 (electronic bk.). - ISBN 1789952107 (electronic bk.)
Description based on online resource; title from copyright page (Safari, viewed May 15, 2019).
Рубрики: Marketing--Data processing.
Marketing research.
Python (Computer program language)
Information visualization.
BUSINESS & ECONOMICS / Industrial Management
BUSINESS & ECONOMICS / Management
BUSINESS & ECONOMICS / Management Science
BUSINESS & ECONOMICS / Organizational Behavior
Аннотация: Explore new and more sophisticated tools that reduce your marketing analytics efforts and give you precise results Key Features Study new techniques for marketing analytics Explore uses of machine learning to power your marketing analyses Work through each stage of data analytics with the help of multiple examples and exercises Book Description Data Science for Marketing Analytics covers every stage of data analytics, from working with a raw dataset to segmenting a population and modeling different parts of the population based on the segments. The book starts by teaching you how to use Python libraries, such as pandas and Matplotlib, to read data from Python, manipulate it, and create plots, using both categorical and continuous variables. Then, you'll learn how to segment a population into groups and use different clustering techniques to evaluate customer segmentation. As you make your way through the chapters, you'll explore ways to evaluate and select the best segmentation approach, and go on to create a linear regression model on customer value data to predict lifetime value. In the concluding chapters, you'll gain an understanding of regression techniques and tools for evaluating regression models, and explore ways to predict customer choice using classification algorithms. Finally, you'll apply these techniques to create a churn model for modeling customer product choices. By the end of this book, you will be able to build your own marketing reporting and interactive dashboard solutions. What you will learn Analyze and visualize data in Python using pandas and Matplotlib Study clustering techniques, such as hierarchical and k-means clustering Create customer segments based on manipulated data Predict customer lifetime value using linear regression Use classification algorithms to understand customer choice Optimize classification algorithms to extract maximal information Who this book is for Data Science for Marketing Analytics is designed for developers and marketing analysts looking to use new, more sophisticated tools in their marketing analytics efforts. It'll help if you have prior experience of coding in Python and knowledge of high school level mathematics. Some experience with databases, Excel, statistics, or Tableau is useful but not necessary. Downloading the example code for this book You can download the example code files for all Packt books you have purchased from your account at http://www.PacktPub.com. If you purchased ...
Доп.точки доступа:
Bhatnagar, Pranshu, \author.\
Behera, Debasish, \author.\
2.
Подробнее
DDC 658.8/7
P 91
Predicting trends and building strategies for consumer engagement in retail environments / / Giuseppe Granata, Andrea Moretta Tartaglione, and Theodosios Tsiakis, editors. - Hershey, PA : : Business Science Reference,, [2019]. - 1 online resource. - URL: https://library.dvfu.ru/lib/document/SK_ELIB/AC4F48FB-8C3A-4716-9120-93F0F202D69F. - ISBN 9781522578574 (electronic bk.). - ISBN 1522578579 (electronic bk.)
Print version record.
Параллельные издания: Print version: : Predicting trends and building strategies for consumer engagement in retail environments. - Hershey, PA : Business Science Reference, [2019]. - ISBN 9781522578567
~РУБ DDC 658.8/7
Рубрики: Retail trade.
Consumer behavior.
Marketing research.
Consumer behavior.
Marketing research.
Retail trade.
BUSINESS & ECONOMICS / Industrial Management
BUSINESS & ECONOMICS / Management
BUSINESS & ECONOMICS / Management Science
BUSINESS & ECONOMICS / Organizational Behavior
Аннотация: "This book provides theoretical and practical insights to improve the understanding of retail complex environments and consumers' shopping behaviors thus enabling the prediction of trends and the elaboration of strategies for retail practitioners in the time of retailers' crisis"--
Доп.точки доступа:
Granata, Giuseppe, (1965-) \editor.\
Tartaglione, Andrea Moretta, (1971-) \editor.\
Tsiakis, Theodosios, (1978-) \editor.\
P 91
Predicting trends and building strategies for consumer engagement in retail environments / / Giuseppe Granata, Andrea Moretta Tartaglione, and Theodosios Tsiakis, editors. - Hershey, PA : : Business Science Reference,, [2019]. - 1 online resource. - URL: https://library.dvfu.ru/lib/document/SK_ELIB/AC4F48FB-8C3A-4716-9120-93F0F202D69F. - ISBN 9781522578574 (electronic bk.). - ISBN 1522578579 (electronic bk.)
Print version record.
Параллельные издания: Print version: : Predicting trends and building strategies for consumer engagement in retail environments. - Hershey, PA : Business Science Reference, [2019]. - ISBN 9781522578567
Рубрики: Retail trade.
Consumer behavior.
Marketing research.
Consumer behavior.
Marketing research.
Retail trade.
BUSINESS & ECONOMICS / Industrial Management
BUSINESS & ECONOMICS / Management
BUSINESS & ECONOMICS / Management Science
BUSINESS & ECONOMICS / Organizational Behavior
Аннотация: "This book provides theoretical and practical insights to improve the understanding of retail complex environments and consumers' shopping behaviors thus enabling the prediction of trends and the elaboration of strategies for retail practitioners in the time of retailers' crisis"--
Доп.точки доступа:
Granata, Giuseppe, (1965-) \editor.\
Tartaglione, Andrea Moretta, (1971-) \editor.\
Tsiakis, Theodosios, (1978-) \editor.\
3.
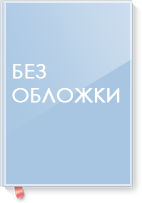
Подробнее
DDC 658.834
H 98
Hwang, Yoon Hyup,.
Hands-on data science for marketing : : improve your marketing strategies with machine learning using Python and R / / Yoon Hyup Hwang. - Birmingham, UK : : Packt Publishing,, 2019. - 1 online resource : : il. - URL: https://library.dvfu.ru/lib/document/SK_ELIB/F02C8E37-4A0A-4335-8AC2-310A4F5C8124. - ISBN 178934882X. - ISBN 9781789348828 (electronic bk.)
Online resource; title from title page (Safari, viewed May 1, 2019).
Параллельные издания: Print version: : Hwang, Yoon Hyup. Hands-On Data Science for Marketing : Improve Your Marketing Strategies with Machine Learning Using Python and R. - Birmingham : Packt Publishing Ltd, ©2019. - ISBN 9781789346343
Содержание:
Cover; Title Page; Copyright and Credits; About Packt; Contributors; Table of Contents; Preface; Section 1: Introduction and Environment Setup; Chapter 1: Data Science and Marketing; Technical requirements; Trends in marketing; Applications of data science in marketing; Descriptive versus explanatory versus predictive analyses; Types of learning algorithms; Data science workflow; Setting up the Python environment; Installing the Anaconda distribution; A simple logistic regression model in Python; Setting up the R environment; Installing R and RStudio; A simple logistic regression model in R
Chapter 3: Drivers behind Marketing EngagementUsing regression analysis for explanatory analysis; Explanatory analysis and regression analysis; Logistic regression; Regression analysis with Python; Data analysis and visualizations; Engagement rate; Sales channels; Total claim amounts; Regression analysis; Continuous variables; Categorical variables; Combining continuous and categorical variables; Regression analysis with R; Data analysis and visualization; Engagement rate; Sales channels; Total claim amounts; Regression analysis; Continuous variables; Categorical variables
Combining continuous and categorical variablesSummary; Chapter 4: From Engagement to Conversion; Decision trees; Logistic regression versus decision trees; Growing decision trees; Decision trees and interpretations with Python; Data analysis and visualization; Conversion rate; Conversion rates by job; Default rates by conversions; Bank balances by conversions; Conversion rates by number of contacts; Encoding categorical variables; Encoding months; Encoding jobs; Encoding marital; Encoding the housing and loan variables; Building decision trees; Interpreting decision trees
Decision trees and interpretations with RData analysis and visualizations; Conversion rate; Conversion rates by job; Default rates by conversions; Bank balance by conversions; Conversion rates by number of contacts; Encoding categorical variables; Encoding the month; Encoding the job, housing, and marital variables; Building decision trees; Interpreting decision trees; Summary; Section 3: Product Visibility and Marketing; Chapter 5: Product Analytics; The importance of product analytics; Product analytics using Python; Time series trends; Repeat customers; Trending items over time
~РУБ DDC 658.834
Рубрики: Marketing--Data processing.
Machine learning.
Marketing research.
Python (Computer program language)
R (Computer program language)
Machine learning.
Marketing--Data processing.
Marketing research.
Python (Computer program language)
R (Computer program language)
Аннотация: Section 2: Descriptive Versus Explanatory Analysis; Chapter 2: Key Performance Indicators and Visualizations; KPIs to measure performances of different marketing efforts; Sales revenue; Cost per acquisition (CPA); Digital marketing KPIs; Computing and visualizing KPIs using Python; Aggregate conversion rate; Conversion rates by age; Conversions versus non-conversions; Conversions by age and marital status; Computing and visualizing KPIs using R; Aggregate conversion rate; Conversion rates by age; Conversions versus non-conversions; Conversions by age and marital status; Summary
This book will be an excellent resource for both Python and R developers and will help them apply data science and machine learning to marketing with real-world data sets. By the end of this book, you will be well equipped with the required knowledge and expertise to draw insights from data and improve your marketing strategies.
H 98
Hwang, Yoon Hyup,.
Hands-on data science for marketing : : improve your marketing strategies with machine learning using Python and R / / Yoon Hyup Hwang. - Birmingham, UK : : Packt Publishing,, 2019. - 1 online resource : : il. - URL: https://library.dvfu.ru/lib/document/SK_ELIB/F02C8E37-4A0A-4335-8AC2-310A4F5C8124. - ISBN 178934882X. - ISBN 9781789348828 (electronic bk.)
Online resource; title from title page (Safari, viewed May 1, 2019).
Параллельные издания: Print version: : Hwang, Yoon Hyup. Hands-On Data Science for Marketing : Improve Your Marketing Strategies with Machine Learning Using Python and R. - Birmingham : Packt Publishing Ltd, ©2019. - ISBN 9781789346343
Содержание:
Cover; Title Page; Copyright and Credits; About Packt; Contributors; Table of Contents; Preface; Section 1: Introduction and Environment Setup; Chapter 1: Data Science and Marketing; Technical requirements; Trends in marketing; Applications of data science in marketing; Descriptive versus explanatory versus predictive analyses; Types of learning algorithms; Data science workflow; Setting up the Python environment; Installing the Anaconda distribution; A simple logistic regression model in Python; Setting up the R environment; Installing R and RStudio; A simple logistic regression model in R
Chapter 3: Drivers behind Marketing EngagementUsing regression analysis for explanatory analysis; Explanatory analysis and regression analysis; Logistic regression; Regression analysis with Python; Data analysis and visualizations; Engagement rate; Sales channels; Total claim amounts; Regression analysis; Continuous variables; Categorical variables; Combining continuous and categorical variables; Regression analysis with R; Data analysis and visualization; Engagement rate; Sales channels; Total claim amounts; Regression analysis; Continuous variables; Categorical variables
Combining continuous and categorical variablesSummary; Chapter 4: From Engagement to Conversion; Decision trees; Logistic regression versus decision trees; Growing decision trees; Decision trees and interpretations with Python; Data analysis and visualization; Conversion rate; Conversion rates by job; Default rates by conversions; Bank balances by conversions; Conversion rates by number of contacts; Encoding categorical variables; Encoding months; Encoding jobs; Encoding marital; Encoding the housing and loan variables; Building decision trees; Interpreting decision trees
Decision trees and interpretations with RData analysis and visualizations; Conversion rate; Conversion rates by job; Default rates by conversions; Bank balance by conversions; Conversion rates by number of contacts; Encoding categorical variables; Encoding the month; Encoding the job, housing, and marital variables; Building decision trees; Interpreting decision trees; Summary; Section 3: Product Visibility and Marketing; Chapter 5: Product Analytics; The importance of product analytics; Product analytics using Python; Time series trends; Repeat customers; Trending items over time
Рубрики: Marketing--Data processing.
Machine learning.
Marketing research.
Python (Computer program language)
R (Computer program language)
Machine learning.
Marketing--Data processing.
Marketing research.
Python (Computer program language)
R (Computer program language)
Аннотация: Section 2: Descriptive Versus Explanatory Analysis; Chapter 2: Key Performance Indicators and Visualizations; KPIs to measure performances of different marketing efforts; Sales revenue; Cost per acquisition (CPA); Digital marketing KPIs; Computing and visualizing KPIs using Python; Aggregate conversion rate; Conversion rates by age; Conversions versus non-conversions; Conversions by age and marital status; Computing and visualizing KPIs using R; Aggregate conversion rate; Conversion rates by age; Conversions versus non-conversions; Conversions by age and marital status; Summary
This book will be an excellent resource for both Python and R developers and will help them apply data science and machine learning to marketing with real-world data sets. By the end of this book, you will be well equipped with the required knowledge and expertise to draw insights from data and improve your marketing strategies.
Страница 1, Результатов: 3