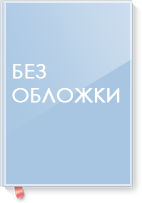
> DDC 006.3015
F 25
Farrell, Peter, (1966-).
The statistics and calculus workshop : a comprehensive introduction to mathematics in Python for artificial intelligence applications / / Peter Farrell [and five others]. - Birmingham : : Packt Publishing,, 2020. - 1 online resource. - URL: https://library.dvfu.ru/lib/document/SK_ELIB/5349CE3F-94F3-473E-94B8-E891ED2935F4
. - ISBN 9781800208360 (electronic book). - ISBN 1800208367 (electronic book)
Параллельные издания: Print version: :
Содержание:
Cover -- FM -- Copyright -- Table of Contents -- Preface -- Chapter 1: Fundamentals of Python -- Introduction -- Control Flow Methods -- if Statements -- Exercise 1.01: Divisibility with Conditionals -- Loops -- The while Loop -- The for Loop -- Exercise 1.02: Number Guessing Game -- Data Structures -- Strings -- Lists -- Exercise 1.03: Multi-Dimensional Lists -- Tuples -- Sets -- Dictionaries -- Exercise 1.04: Shopping Cart Calculations -- Functions and Algorithms -- Functions -- Exercise 1.05: Finding the Maximum -- Recursion -- Exercise 1.06: The Tower of Hanoi -- Algorithm Design
Exercise 1.07: The N-Queens Problem -- Testing, Debugging, and Version Control -- Testing -- Debugging -- Exercise 1.08: Testing for Concurrency -- Version Control -- Exercise 1.09: Version Control with Git and GitHub -- Activity 1.01: Building a Sudoku Solver -- Summary -- Chapter 2: Python's Main Tools for Statistics -- Introduction -- Scientific Computing and NumPy Basics -- NumPy Arrays -- Vectorization -- Exercise 2.01: Timing Vectorized Operations in NumPy -- Random Sampling -- Working with Tabular Data in pandas -- Initializing a DataFrame Object -- Accessing Rows and Columns
Manipulating DataFrames -- Exercise 2.02: Data Table Manipulation -- Advanced Pandas Functionalities -- Exercise 2.03: The Student Dataset -- Data Visualization with Matplotlib and Seaborn -- Scatter Plots -- Line Graphs -- Bar Graphs -- Histograms -- Heatmaps -- Exercise 2.04: Visualization of Probability Distributions -- Visualization Shorthand from Seaborn and Pandas -- Activity 2.01: Analyzing the Communities and Crime Dataset -- Summary -- Chapter 3: Python's Statistical Toolbox -- Introduction -- An Overview of Statistics -- Types of Data in Statistics -- Categorical Data
Exercise 3.01: Visualizing Weather Percentages -- Numerical Data -- Exercise 3.02: Min-Max Scaling -- Ordinal Data -- Descriptive Statistics -- Central Tendency -- Dispersion -- Exercise 3.03: Visualizing Probability Density Functions -- Python-Related Descriptive Statistics -- Inferential Statistics -- T-Tests -- Correlation Matrix -- Exercise 3.04: Identifying and Testing Equality of Means -- Statistical and Machine Learning Models -- Exercise 3.05: Model Selection -- Python's Other Statistics Tools -- Activity 3.01: Revisiting the Communities and Crimes Dataset -- Summary
Chapter 4: Functions and Algebra with Python -- Introduction -- Functions -- Common Functions -- Domain and Range -- Function Roots and Equations -- The Plot of a Function -- Exercise 4.01: Function Identification from Plots -- Function Transformations -- Shifts -- Scaling -- Exercise 4.02: Function Transformation Identification -- Equations -- Algebraic Manipulations -- Factoring -- Using Python -- Exercise 4.03: Introduction to Break-Even Analysis -- Systems of Equations -- Systems of Linear Equations -- Exercise 4.04: Matrix Solution with NumPy -- Systems of Non-Linear Equations
Рубрики: Artificial intelligence--Mathematics.
Python (Computer program language)
Intelligence artificielle--Mathématiques.
Python (Langage de programmation)
Artificial intelligence--Mathematics
Python (Computer program language)
Аннотация: With examples and activities that help you achieve real results, applying calculus and statistical methods relevant to advanced data science has never been so easy Key Features Discover how most programmers use the main Python libraries when performing statistics with Python Use descriptive statistics and visualizations to answer business and scientific questions Solve complicated calculus problems, such as arc length and solids of revolution using derivatives and integrals Book Description Are you looking to start developing artificial intelligence applications? Do you need a refresher on key mathematical concepts? Full of engaging practical exercises, The Statistics and Calculus with Python Workshop will show you how to apply your understanding of advanced mathematics in the context of Python. The book begins by giving you a high-level overview of the libraries you'll use while performing statistics with Python. As you progress, you'll perform various mathematical tasks using the Python programming language, such as solving algebraic functions with Python starting with basic functions, and then working through transformations and solving equations. Later chapters in the book will cover statistics and calculus concepts and how to use them to solve problems and gain useful insights. Finally, you'll study differential equations with an emphasis on numerical methods and learn about algorithms that directly calculate values of functions. By the end of this book, you'll have learned how to apply essential statistics and calculus concepts to develop robust Python applications that solve business challenges. What you will learn Get to grips with the fundamental mathematical functions in Python Perform calculations on tabular datasets using pandas Understand the differences between polynomials, rational functions, exponential functions, and trigonometric functions Use algebra techniques for solving systems of equations Solve real-world problems with probability Solve optimization problems with derivatives and integrals Who this book is for If you are a Python programmer who wants to develop intelligent solutions that solve challenging business problems, then this book is for you. To better grasp the concepts explained in this book, you must have a thorough understanding of advanced mathematical concepts, such as Markov chains, Euler's formula, and Runge-Kutta methods as the book only explains how these techniques and concepts can be implemented in Py...